Deeplab的复现(pytorch实现)
DeepLab复现的pytorch实现
本文复现的主要是deeplabv3。使用的数据集和之前发的文章FCN一样,没有了解的可以移步到之前发的文章中去查看一下。
1.该模型的主要结构
对于代码部分,主要只写了模型部分的,其他部分内容基本和FCN的一致,在下面也会给出完整代码仓库的地址方便大家进行学习。
from collections import OrderedDictfrom typing import Dict, Listimport torch
from torch import nn, Tensor
from torch.nn import functional as F
from .resnet_backbone import resnet50, resnet101
from .mobilenet_backbone import mobilenet_v3_largeclass IntermediateLayerGetter(nn.ModuleDict): # 获取模型指定的中间层输出"""Module wrapper that returns intermediate layers from a modelIt has a strong assumption that the modules have been registeredinto the model in the same order as they are used.This means that one should **not** reuse the same nn.Moduletwice in the forward if you want this to work.Additionally, it is only able to query submodules that are directlyassigned to the model. So if `model` is passed, `model.feature1` canbe returned, but not `model.feature1.layer2`.Args:model (nn.Module): model on which we will extract the featuresreturn_layers (Dict[name, new_name]): a dict containing the namesof the modules for which the activations will be returned asthe key of the dict, and the value of the dict is the nameof the returned activation (which the user can specify)."""_version = 2__annotations__ = {"return_layers": Dict[str, str],}def __init__(self, model: nn.Module, return_layers: Dict[str, str]) -> None:if not set(return_layers).issubset([name for name, _ in model.named_children()]):raise ValueError("return_layers are not present in model")orig_return_layers = return_layersreturn_layers = {str(k): str(v) for k, v in return_layers.items()}# 重新构建backbone,将没有使用到的模块全部删掉layers = OrderedDict()for name, module in model.named_children():layers[name] = moduleif name in return_layers:del return_layers[name]if not return_layers:breaksuper(IntermediateLayerGetter, self).__init__(layers)self.return_layers = orig_return_layersdef forward(self, x: Tensor) -> Dict[str, Tensor]:out = OrderedDict()for name, module in self.items():x = module(x)if name in self.return_layers:out_name = self.return_layers[name]out[out_name] = xreturn outclass DeepLabV3(nn.Module):"""Implements DeepLabV3 model from`"Rethinking Atrous Convolution for Semantic Image Segmentation"<https://arxiv.org/abs/1706.05587>`_.Args:backbone (nn.Module): the network used to compute the features for the model.The backbone should return an OrderedDict[Tensor], with the key being"out" for the last feature map used, and "aux" if an auxiliary classifieris used.classifier (nn.Module): module that takes the "out" element returned fromthe backbone and returns a dense prediction.aux_classifier (nn.Module, optional): auxiliary classifier used during training"""__constants__ = ['aux_classifier']def __init__(self, backbone, classifier, aux_classifier=None):super(DeepLabV3, self).__init__()self.backbone = backboneself.classifier = classifierself.aux_classifier = aux_classifierdef forward(self, x: Tensor) -> Dict[str, Tensor]:input_shape = x.shape[-2:]# contract: features is a dict of tensorsfeatures = self.backbone(x)result = OrderedDict()x = features["out"]x = self.classifier(x)# 使用双线性插值还原回原图尺度x = F.interpolate(x, size=input_shape, mode='bilinear', align_corners=False)result["out"] = xif self.aux_classifier is not None:x = features["aux"]x = self.aux_classifier(x)# 使用双线性插值还原回原图尺度x = F.interpolate(x, size=input_shape, mode='bilinear', align_corners=False)result["aux"] = xreturn resultclass FCNHead(nn.Sequential):def __init__(self, in_channels, channels):inter_channels = in_channels // 4 # 两个//表示地板除,即先做除法,然后向下取整super(FCNHead, self).__init__(nn.Conv2d(in_channels, inter_channels, 3, padding=1, bias=False),nn.BatchNorm2d(inter_channels),nn.ReLU(),nn.Dropout(0.1),nn.Conv2d(inter_channels, channels, 1))class ASPPConv(nn.Sequential):def __init__(self, in_channels: int, out_channels: int, dilation: int) -> None:super(ASPPConv, self).__init__(nn.Conv2d(in_channels, out_channels, 3, padding=dilation, dilation=dilation, bias=False),nn.BatchNorm2d(out_channels),nn.ReLU())class ASPPPooling(nn.Sequential):def __init__(self, in_channels: int, out_channels: int) -> None:super(ASPPPooling, self).__init__(nn.AdaptiveAvgPool2d(1),nn.Conv2d(in_channels, out_channels, 1, bias=False),nn.BatchNorm2d(out_channels),nn.ReLU())def forward(self, x: torch.Tensor) -> torch.Tensor:size = x.shape[-2:]for mod in self:x = mod(x)return F.interpolate(x, size=size, mode='bilinear', align_corners=False)class ASPP(nn.Module):def __init__(self, in_channels: int, atrous_rates: List[int], out_channels: int = 256) -> None:super(ASPP, self).__init__()modules = [nn.Sequential(nn.Conv2d(in_channels, out_channels, 1, bias=False),nn.BatchNorm2d(out_channels),nn.ReLU())]rates = tuple(atrous_rates)for rate in rates:modules.append(ASPPConv(in_channels, out_channels, rate))modules.append(ASPPPooling(in_channels, out_channels))self.convs = nn.ModuleList(modules)self.project = nn.Sequential(nn.Conv2d(len(self.convs) * out_channels, out_channels, 1, bias=False),nn.BatchNorm2d(out_channels),nn.ReLU(),nn.Dropout(0.5))def forward(self, x: torch.Tensor) -> torch.Tensor:_res = []for conv in self.convs:_res.append(conv(x))res = torch.cat(_res, dim=1)return self.project(res)class DeepLabHead(nn.Sequential):def __init__(self, in_channels: int, num_classes: int) -> None:super(DeepLabHead, self).__init__(ASPP(in_channels, [12, 24, 36]),nn.Conv2d(256, 256, 3, padding=1, bias=False),nn.BatchNorm2d(256),nn.ReLU(),nn.Conv2d(256, num_classes, 1))def deeplabv3_resnet50(aux, num_classes=21, pretrain_backbone=False):# 'resnet50_imagenet': 'https://download.pytorch.org/models/resnet50-0676ba61.pth'# 'deeplabv3_resnet50_coco': 'https://download.pytorch.org/models/deeplabv3_resnet50_coco-cd0a2569.pth'backbone = resnet50(replace_stride_with_dilation=[False, True, True])if pretrain_backbone:# 载入resnet50 backbone预训练权重backbone.load_state_dict(torch.load("resnet50.pth", map_location='cpu'))out_inplanes = 2048aux_inplanes = 1024return_layers = {'layer4': 'out'}if aux:return_layers['layer3'] = 'aux'backbone = IntermediateLayerGetter(backbone, return_layers=return_layers)aux_classifier = None# why using aux: https://github.com/pytorch/vision/issues/4292if aux:aux_classifier = FCNHead(aux_inplanes, num_classes)classifier = DeepLabHead(out_inplanes, num_classes)model = DeepLabV3(backbone, classifier, aux_classifier)return modeldef deeplabv3_resnet101(aux, num_classes=21, pretrain_backbone=False):# 'resnet101_imagenet': 'https://download.pytorch.org/models/resnet101-63fe2227.pth'# 'deeplabv3_resnet101_coco': 'https://download.pytorch.org/models/deeplabv3_resnet101_coco-586e9e4e.pth'backbone = resnet101(replace_stride_with_dilation=[False, True, True])if pretrain_backbone:# 载入resnet101 backbone预训练权重backbone.load_state_dict(torch.load("resnet101.pth", map_location='cpu'))out_inplanes = 2048aux_inplanes = 1024return_layers = {'layer4': 'out'}if aux:return_layers['layer3'] = 'aux'backbone = IntermediateLayerGetter(backbone, return_layers=return_layers)aux_classifier = None# why using aux: https://github.com/pytorch/vision/issues/4292if aux:aux_classifier = FCNHead(aux_inplanes, num_classes)classifier = DeepLabHead(out_inplanes, num_classes)model = DeepLabV3(backbone, classifier, aux_classifier)return modeldef deeplabv3_mobilenetv3_large(aux, num_classes=21, pretrain_backbone=False):# 'mobilenetv3_large_imagenet': 'https://download.pytorch.org/models/mobilenet_v3_large-8738ca79.pth'# 'depv3_mobilenetv3_large_coco': "https://download.pytorch.org/models/deeplabv3_mobilenet_v3_large-fc3c493d.pth"backbone = mobilenet_v3_large(dilated=True)if pretrain_backbone:# 载入mobilenetv3 large backbone预训练权重backbone.load_state_dict(torch.load("mobilenet_v3_large.pth", map_location='cpu'))backbone = backbone.features# Gather the indices of blocks which are strided. These are the locations of C1, ..., Cn-1 blocks.# The first and last blocks are always included because they are the C0 (conv1) and Cn.stage_indices = [0] + [i for i, b in enumerate(backbone) if getattr(b, "is_strided", False)] + [len(backbone) - 1]out_pos = stage_indices[-1] # use C5 which has output_stride = 16out_inplanes = backbone[out_pos].out_channelsaux_pos = stage_indices[-4] # use C2 here which has output_stride = 8aux_inplanes = backbone[aux_pos].out_channelsreturn_layers = {str(out_pos): "out"}if aux:return_layers[str(aux_pos)] = "aux"backbone = IntermediateLayerGetter(backbone, return_layers=return_layers)aux_classifier = None# why using aux: https://github.com/pytorch/vision/issues/4292if aux:aux_classifier = FCNHead(aux_inplanes, num_classes)classifier = DeepLabHead(out_inplanes, num_classes)model = DeepLabV3(backbone, classifier, aux_classifier)return model----------------------------------------------------------------------------------分割线-------------------------------------------from typing import Callable, List, Optionalimport torch
from torch import nn, Tensor
from torch.nn import functional as F
from functools import partialdef _make_divisible(ch, divisor=8, min_ch=None): # 为了使每一层的通道数都可以被8整除"""This function is taken from the original tf repo.It ensures that all layers have a channel number that is divisible by 8It can be seen here:https://github.com/tensorflow/models/blob/master/research/slim/nets/mobilenet/mobilenet.py"""if min_ch is None:min_ch = divisornew_ch = max(min_ch, int(ch + divisor / 2) // divisor * divisor)# Make sure that round down does not go down by more than 10%.if new_ch < 0.9 * ch:new_ch += divisorreturn new_chclass ConvBNActivation(nn.Sequential):def __init__(self,in_planes: int,out_planes: int,kernel_size: int = 3,stride: int = 1,groups: int = 1,norm_layer: Optional[Callable[..., nn.Module]] = None,activation_layer: Optional[Callable[..., nn.Module]] = None,dilation: int = 1):padding = (kernel_size - 1) // 2 * dilationif norm_layer is None:norm_layer = nn.BatchNorm2dif activation_layer is None:activation_layer = nn.ReLU6super(ConvBNActivation, self).__init__(nn.Conv2d(in_channels=in_planes,out_channels=out_planes,kernel_size=kernel_size,stride=stride,dilation=dilation,padding=padding,groups=groups,bias=False),norm_layer(out_planes),activation_layer(inplace=True))self.out_channels = out_planesclass SqueezeExcitation(nn.Module):def __init__(self, input_c: int, squeeze_factor: int = 4):super(SqueezeExcitation, self).__init__()squeeze_c = _make_divisible(input_c // squeeze_factor, 8)self.fc1 = nn.Conv2d(input_c, squeeze_c, 1)self.fc2 = nn.Conv2d(squeeze_c, input_c, 1)def forward(self, x: Tensor) -> Tensor:scale = F.adaptive_avg_pool2d(x, output_size=(1, 1))scale = self.fc1(scale)scale = F.relu(scale, inplace=True)scale = self.fc2(scale)scale = F.hardsigmoid(scale, inplace=True)return scale * xclass InvertedResidualConfig:def __init__(self,input_c: int,kernel: int,expanded_c: int,out_c: int,use_se: bool,activation: str,stride: int,dilation: int,width_multi: float):self.input_c = self.adjust_channels(input_c, width_multi)self.kernel = kernelself.expanded_c = self.adjust_channels(expanded_c, width_multi)self.out_c = self.adjust_channels(out_c, width_multi)self.use_se = use_seself.use_hs = activation == "HS" # whether using h-swish activationself.stride = strideself.dilation = dilation@staticmethoddef adjust_channels(channels: int, width_multi: float):return _make_divisible(channels * width_multi, 8)class InvertedResidual(nn.Module):def __init__(self,cnf: InvertedResidualConfig,norm_layer: Callable[..., nn.Module]):super(InvertedResidual, self).__init__()if cnf.stride not in [1, 2]:raise ValueError("illegal stride value.")self.use_res_connect = (cnf.stride == 1 and cnf.input_c == cnf.out_c)layers: List[nn.Module] = []activation_layer = nn.Hardswish if cnf.use_hs else nn.ReLU# expandif cnf.expanded_c != cnf.input_c:layers.append(ConvBNActivation(cnf.input_c,cnf.expanded_c,kernel_size=1,norm_layer=norm_layer,activation_layer=activation_layer))# depthwisestride = 1 if cnf.dilation > 1 else cnf.stridelayers.append(ConvBNActivation(cnf.expanded_c,cnf.expanded_c,kernel_size=cnf.kernel,stride=stride,dilation=cnf.dilation,groups=cnf.expanded_c,norm_layer=norm_layer,activation_layer=activation_layer))if cnf.use_se:layers.append(SqueezeExcitation(cnf.expanded_c))# projectlayers.append(ConvBNActivation(cnf.expanded_c,cnf.out_c,kernel_size=1,norm_layer=norm_layer,activation_layer=nn.Identity))self.block = nn.Sequential(*layers)self.out_channels = cnf.out_cself.is_strided = cnf.stride > 1def forward(self, x: Tensor) -> Tensor:result = self.block(x)if self.use_res_connect:result += xreturn resultclass MobileNetV3(nn.Module):def __init__(self,inverted_residual_setting: List[InvertedResidualConfig],last_channel: int,num_classes: int = 1000,block: Optional[Callable[..., nn.Module]] = None,norm_layer: Optional[Callable[..., nn.Module]] = None):super(MobileNetV3, self).__init__()if not inverted_residual_setting:raise ValueError("The inverted_residual_setting should not be empty.")elif not (isinstance(inverted_residual_setting, List) andall([isinstance(s, InvertedResidualConfig) for s in inverted_residual_setting])):raise TypeError("The inverted_residual_setting should be List[InvertedResidualConfig]")if block is None:block = InvertedResidualif norm_layer is None:norm_layer = partial(nn.BatchNorm2d, eps=0.001, momentum=0.01)layers: List[nn.Module] = []# building first layerfirstconv_output_c = inverted_residual_setting[0].input_clayers.append(ConvBNActivation(3,firstconv_output_c,kernel_size=3,stride=2,norm_layer=norm_layer,activation_layer=nn.Hardswish))# building inverted residual blocksfor cnf in inverted_residual_setting:layers.append(block(cnf, norm_layer))# building last several layerslastconv_input_c = inverted_residual_setting[-1].out_clastconv_output_c = 6 * lastconv_input_clayers.append(ConvBNActivation(lastconv_input_c,lastconv_output_c,kernel_size=1,norm_layer=norm_layer,activation_layer=nn.Hardswish))self.features = nn.Sequential(*layers)self.avgpool = nn.AdaptiveAvgPool2d(1)self.classifier = nn.Sequential(nn.Linear(lastconv_output_c, last_channel),nn.Hardswish(inplace=True),nn.Dropout(p=0.2, inplace=True),nn.Linear(last_channel, num_classes))# initial weightsfor m in self.modules():if isinstance(m, nn.Conv2d):nn.init.kaiming_normal_(m.weight, mode="fan_out")if m.bias is not None:nn.init.zeros_(m.bias)elif isinstance(m, (nn.BatchNorm2d, nn.GroupNorm)):nn.init.ones_(m.weight)nn.init.zeros_(m.bias)elif isinstance(m, nn.Linear):nn.init.normal_(m.weight, 0, 0.01)nn.init.zeros_(m.bias)def _forward_impl(self, x: Tensor) -> Tensor:x = self.features(x)x = self.avgpool(x)x = torch.flatten(x, 1)x = self.classifier(x)return xdef forward(self, x: Tensor) -> Tensor:return self._forward_impl(x)def mobilenet_v3_large(num_classes: int = 1000,reduced_tail: bool = False,dilated: bool = False) -> MobileNetV3:"""Constructs a large MobileNetV3 architecture from"Searching for MobileNetV3" <https://arxiv.org/abs/1905.02244>.weights_link:https://download.pytorch.org/models/mobilenet_v3_large-8738ca79.pthArgs:num_classes (int): number of classesreduced_tail (bool): If True, reduces the channel counts of all feature layersbetween C4 and C5 by 2. It is used to reduce the channel redundancy in thebackbone for Detection and Segmentation.dilated: whether using dilated conv"""width_multi = 1.0bneck_conf = partial(InvertedResidualConfig, width_multi=width_multi)adjust_channels = partial(InvertedResidualConfig.adjust_channels, width_multi=width_multi)reduce_divider = 2 if reduced_tail else 1dilation = 2 if dilated else 1inverted_residual_setting = [# input_c, kernel, expanded_c, out_c, use_se, activation, stride, dilationbneck_conf(16, 3, 16, 16, False, "RE", 1, 1),bneck_conf(16, 3, 64, 24, False, "RE", 2, 1), # C1bneck_conf(24, 3, 72, 24, False, "RE", 1, 1),bneck_conf(24, 5, 72, 40, True, "RE", 2, 1), # C2bneck_conf(40, 5, 120, 40, True, "RE", 1, 1),bneck_conf(40, 5, 120, 40, True, "RE", 1, 1),bneck_conf(40, 3, 240, 80, False, "HS", 2, 1), # C3bneck_conf(80, 3, 200, 80, False, "HS", 1, 1),bneck_conf(80, 3, 184, 80, False, "HS", 1, 1),bneck_conf(80, 3, 184, 80, False, "HS", 1, 1),bneck_conf(80, 3, 480, 112, True, "HS", 1, 1),bneck_conf(112, 3, 672, 112, True, "HS", 1, 1),bneck_conf(112, 5, 672, 160 // reduce_divider, True, "HS", 2, dilation), # C4bneck_conf(160 // reduce_divider, 5, 960 // reduce_divider, 160 // reduce_divider, True, "HS", 1, dilation),bneck_conf(160 // reduce_divider, 5, 960 // reduce_divider, 160 // reduce_divider, True, "HS", 1, dilation),]last_channel = adjust_channels(1280 // reduce_divider) # C5return MobileNetV3(inverted_residual_setting=inverted_residual_setting,last_channel=last_channel,num_classes=num_classes)def mobilenet_v3_small(num_classes: int = 1000,reduced_tail: bool = False,dilated: bool = False) -> MobileNetV3:"""Constructs a large MobileNetV3 architecture from"Searching for MobileNetV3" <https://arxiv.org/abs/1905.02244>.weights_link:https://download.pytorch.org/models/mobilenet_v3_small-047dcff4.pthArgs:num_classes (int): number of classesreduced_tail (bool): If True, reduces the channel counts of all feature layersbetween C4 and C5 by 2. It is used to reduce the channel redundancy in thebackbone for Detection and Segmentation.dilated: whether using dilated conv"""width_multi = 1.0bneck_conf = partial(InvertedResidualConfig, width_multi=width_multi)adjust_channels = partial(InvertedResidualConfig.adjust_channels, width_multi=width_multi)reduce_divider = 2 if reduced_tail else 1dilation = 2 if dilated else 1inverted_residual_setting = [# input_c, kernel, expanded_c, out_c, use_se, activation, stride, dilationbneck_conf(16, 3, 16, 16, True, "RE", 2, 1), # C1bneck_conf(16, 3, 72, 24, False, "RE", 2, 1), # C2bneck_conf(24, 3, 88, 24, False, "RE", 1, 1),bneck_conf(24, 5, 96, 40, True, "HS", 2, 1), # C3bneck_conf(40, 5, 240, 40, True, "HS", 1, 1),bneck_conf(40, 5, 240, 40, True, "HS", 1, 1),bneck_conf(40, 5, 120, 48, True, "HS", 1, 1),bneck_conf(48, 5, 144, 48, True, "HS", 1, 1),bneck_conf(48, 5, 288, 96 // reduce_divider, True, "HS", 2, dilation), # C4bneck_conf(96 // reduce_divider, 5, 576 // reduce_divider, 96 // reduce_divider, True, "HS", 1, dilation),bneck_conf(96 // reduce_divider, 5, 576 // reduce_divider, 96 // reduce_divider, True, "HS", 1, dilation)]last_channel = adjust_channels(1024 // reduce_divider) # C5return MobileNetV3(inverted_residual_setting=inverted_residual_setting,last_channel=last_channel,num_classes=num_classes)
在上述代码中,也将之前FCNmodel中没有的mobilenet作为backbone的模型代码也加了上来。
参考链接:
288, 96 // reduce_divider, True, “HS”, 2, dilation), # C4
bneck_conf(96 // reduce_divider, 5, 576 // reduce_divider, 96 // reduce_divider, True, “HS”, 1, dilation),
bneck_conf(96 // reduce_divider, 5, 576 // reduce_divider, 96 // reduce_divider, True, “HS”, 1, dilation)
]
last_channel = adjust_channels(1024 // reduce_divider) # C5
return MobileNetV3(inverted_residual_setting=inverted_residual_setting,last_channel=last_channel,num_classes=num_classes)
在上述代码中,也将之前FCNmodel中没有的mobilenet作为backbone的模型代码也加了上来。参考链接:[deep-learning-for-image-processing/pytorch_segmentation/fcn/src/fcn_model.py at bf4384bfc14e295fdbdc967d6b5093cce0bead17 · WZMIAOMIAO/deep-learning-for-image-processing (github.com)](https://github.com/WZMIAOMIAO/deep-learning-for-image-processing/blob/bf4384bfc14e295fdbdc967d6b5093cce0bead17/pytorch_segmentation/fcn/src/fcn_model.py)
相关文章:
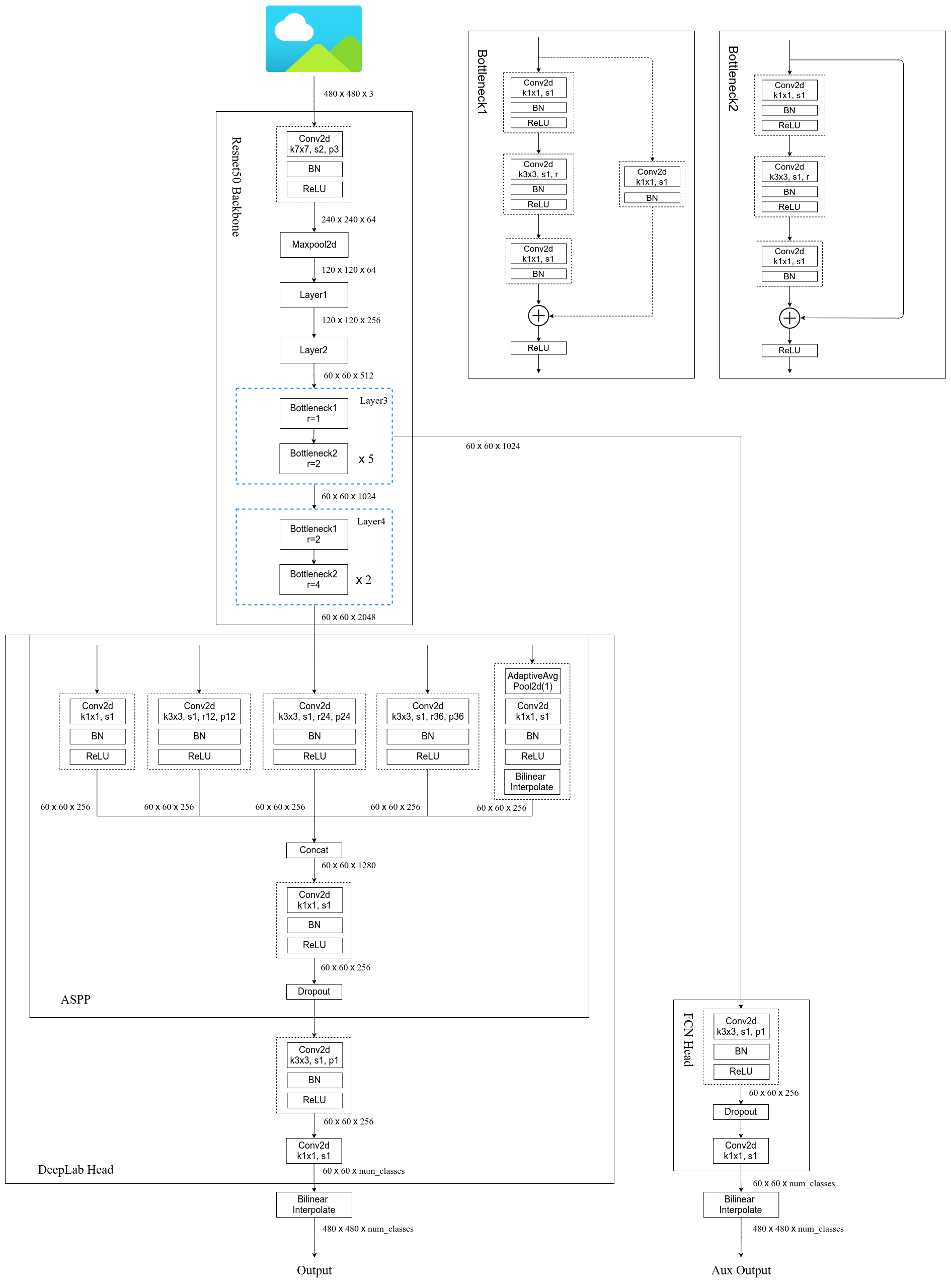
Deeplab的复现(pytorch实现)
DeepLab复现的pytorch实现 本文复现的主要是deeplabv3。使用的数据集和之前发的文章FCN一样,没有了解的可以移步到之前发的文章中去查看一下。 1.该模型的主要结构 对于代码部分,主要只写了模型部分的,其他部分内容基本和FCN的一致…...

input上添加disabled=“true“,点击事件失效处理办法
当我们给input标签上添加disabled"true"时,再添加点击事件,点击事件会不生效,处理办法如下: 给input标签添加样式style"pointer-events: none;" 代码如下: <input style"pointer-event…...
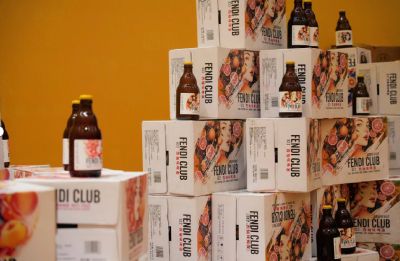
精酿啤酒的魅力:啤酒的与众不同风味
啤酒,作为世界上古老的酒精饮品之一,一直以来都以其与众不同的魅力吸引着无数人的味蕾。而精酿啤酒,作为啤酒中的佼佼者之一,更是以其丰富的口感和多样的风格,成为了啤酒爱好者的心头好。在这其中,Fendi cl…...
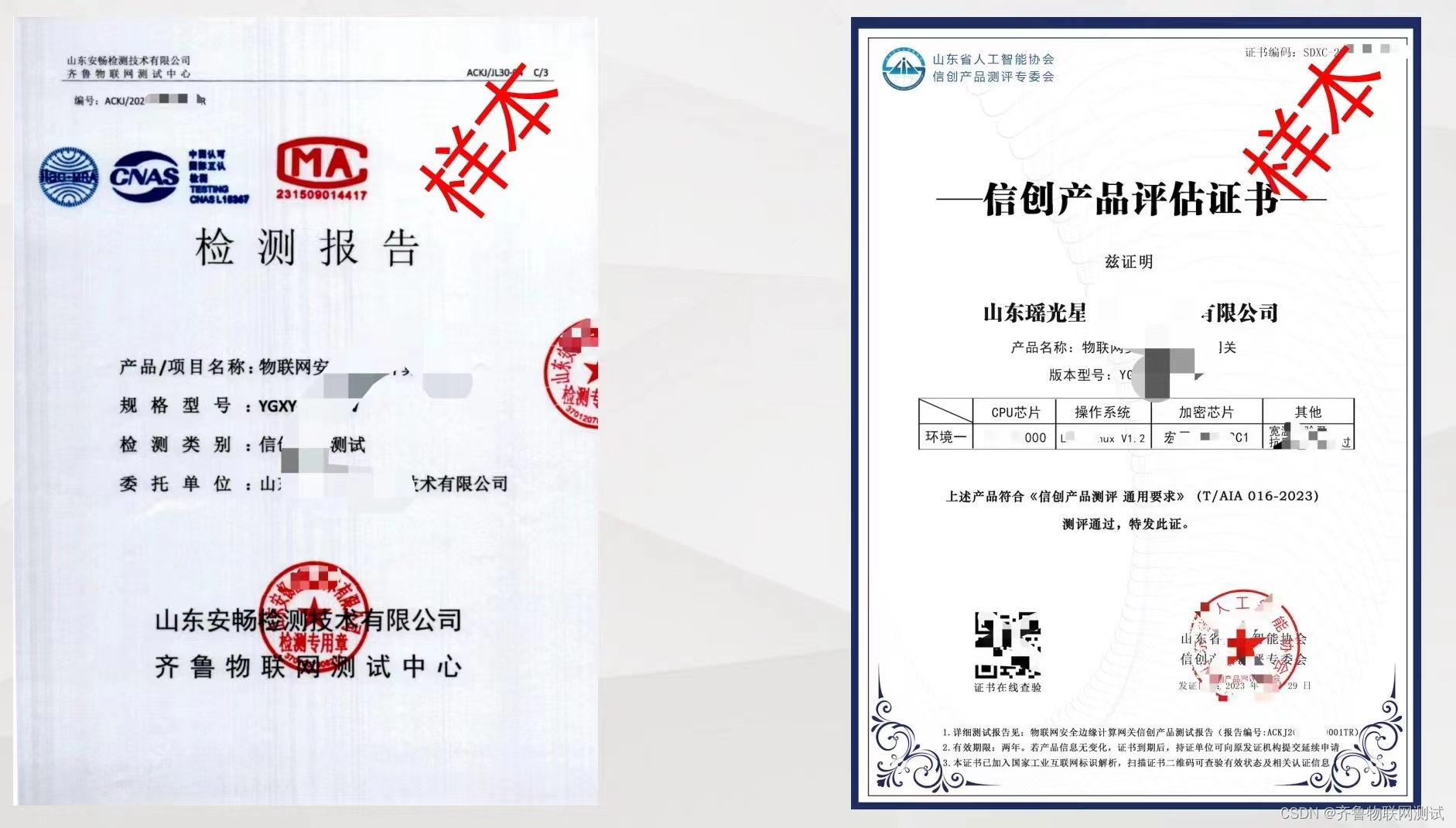
检测机构的双资质是什么?
CMA和CNAS是两种在检测、校准和认证领域具有权威性的资质。 CMA资质全称为“检验检测机构资质认定”(China Inspection Body and Laboratory Mandatory Approval)。它是根据《中华人民共和国计量法》等相关法规,由国家认证认可监督管理委员会…...
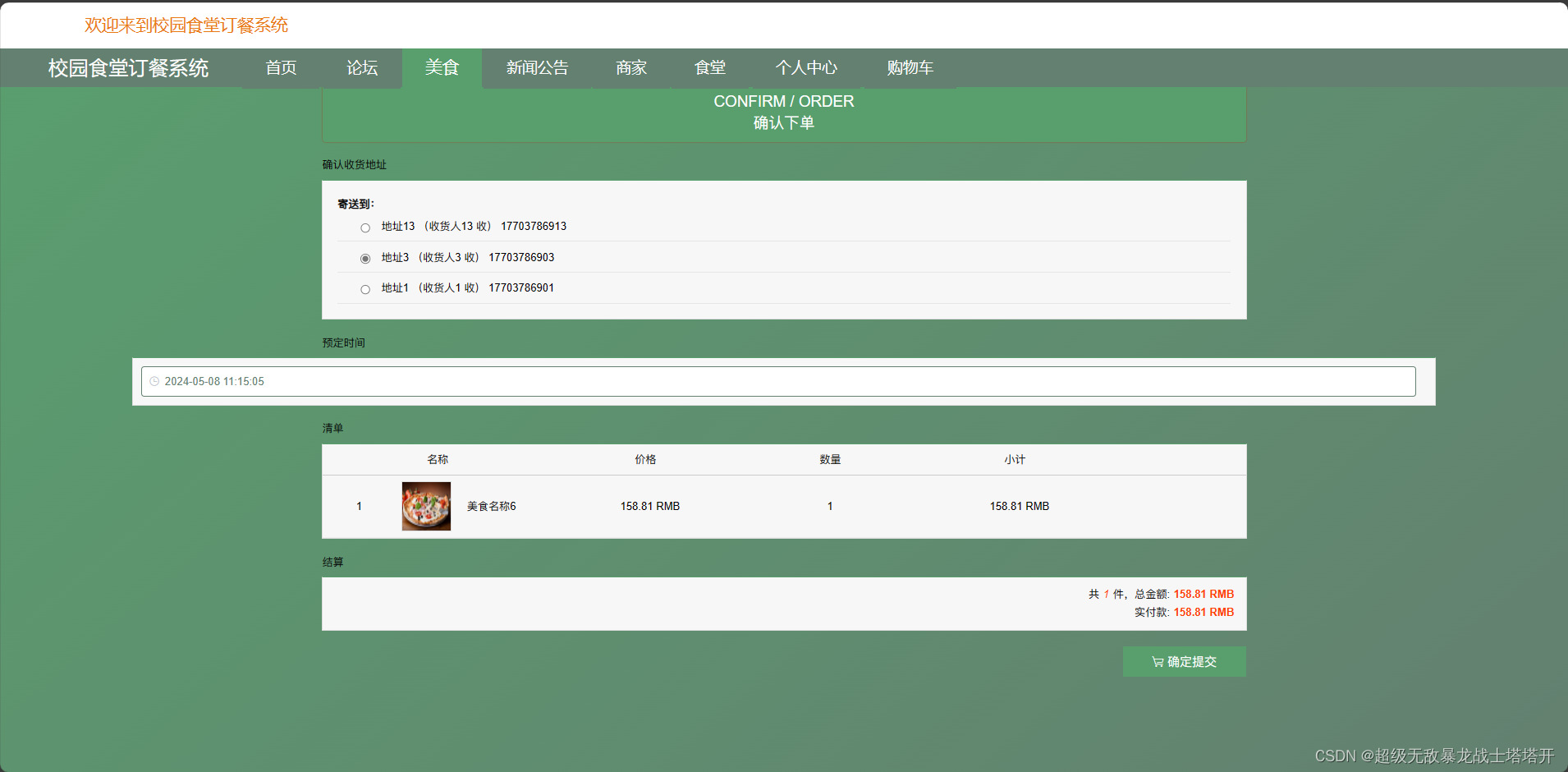
基于springboot的校园食堂订餐系统
文章目录 项目介绍主要功能截图:部分代码展示设计总结项目获取方式 🍅 作者主页:超级无敌暴龙战士塔塔开 🍅 简介:Java领域优质创作者🏆、 简历模板、学习资料、面试题库【关注我,都给你】 &…...
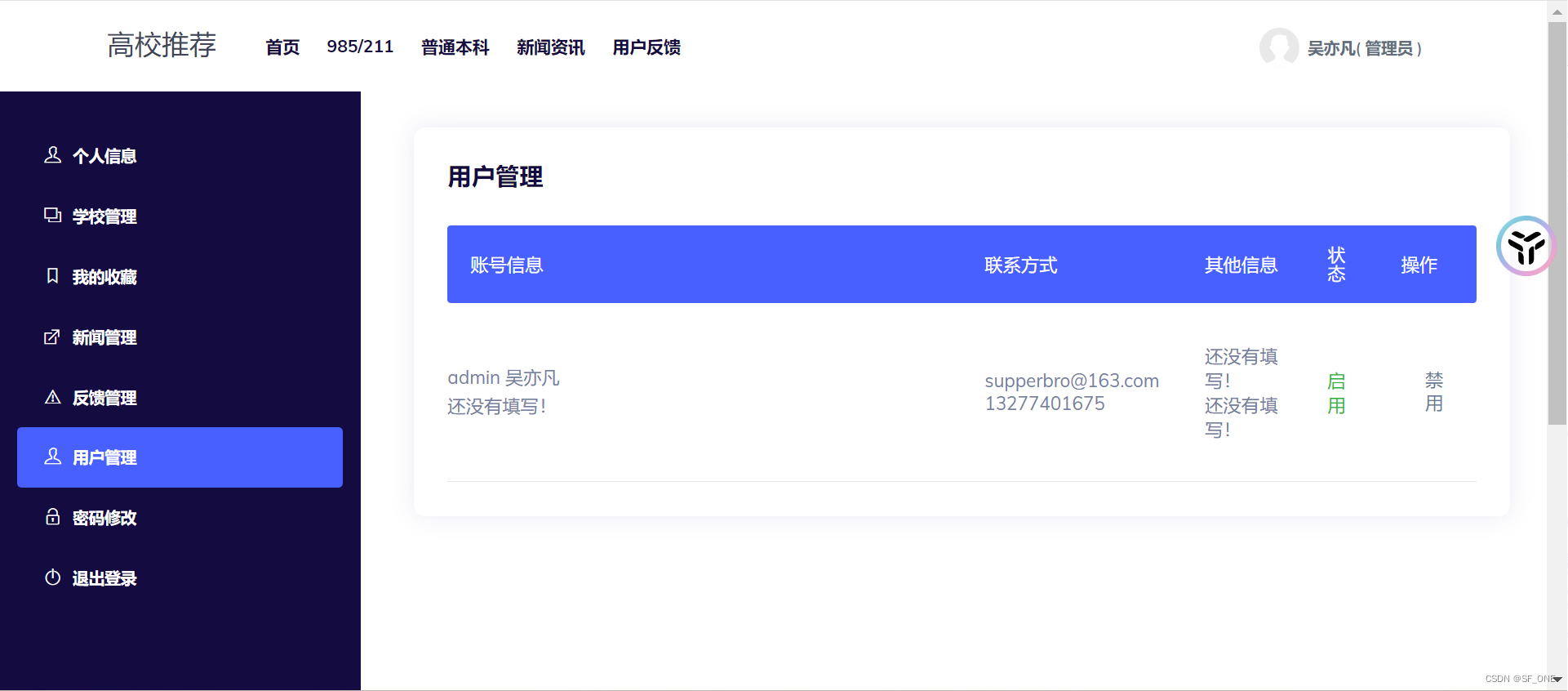
基于SpringBoot的高校推荐系统
项目介绍 当前,随着高等教育的不断普及,越来越多的学生选择考研究生来提高自身的学术水平和竞争力。然而,考研生在选择报考院校和专业时面临着众多的选择和信息不对称的问题。为了解决这些问题,一些网站和APP已经推出了相关的院校…...

了解 websocket
1. 概念 1、 websocket 是一种双向通行协议。实现了浏览器与服务器全双工通信,能更好的节省服务器资源和带宽并达到实时通讯的目的; 2、websocket连接成功后,只要连接不断开,通信就会一保持着; 3、要打开一个 WebS…...

C++中erase函数的用法
在C中,erase函数用于从容器中删除一个或一系列元素。它通常用于删除容器中的指定位置的元素或特定值的元素。 erase函数通常有两种用法: 删除指定位置的元素:erase(iterator position) 这种用法会删除容器中迭代器position指向的元素。 st…...
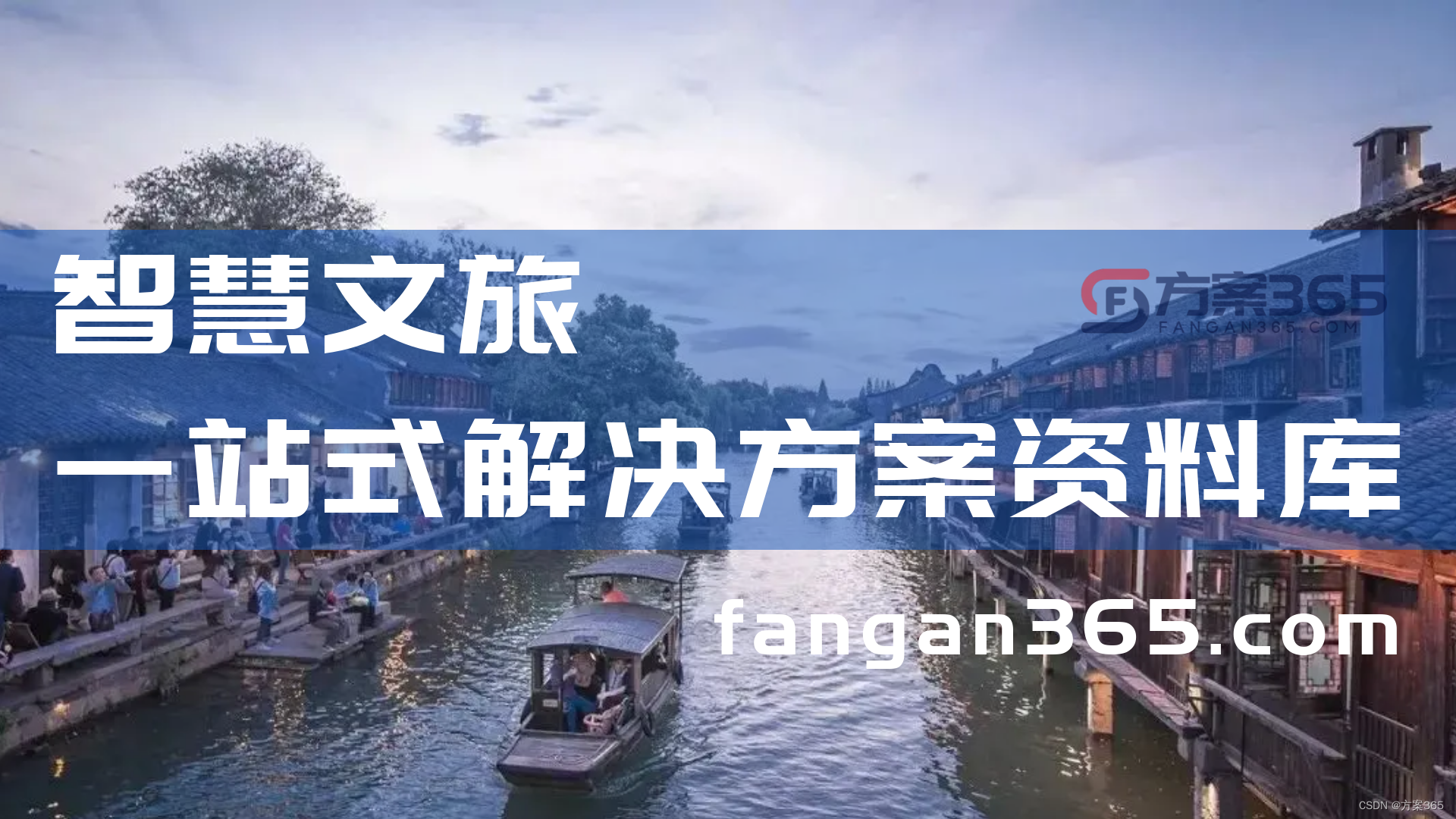
数字旅游以科技创新为核心竞争力:推动旅游服务的智能化、高效化,满足游客日益增长的旅游需求
一、引言 随着科技的飞速发展,数字旅游作为旅游业与信息技术结合的产物,正以其独特的魅力改变着传统旅游业的格局。科技创新作为数字旅游的核心竞争力,不仅推动了旅游服务的智能化、高效化,更满足了游客日益增长的旅游需求。本文…...
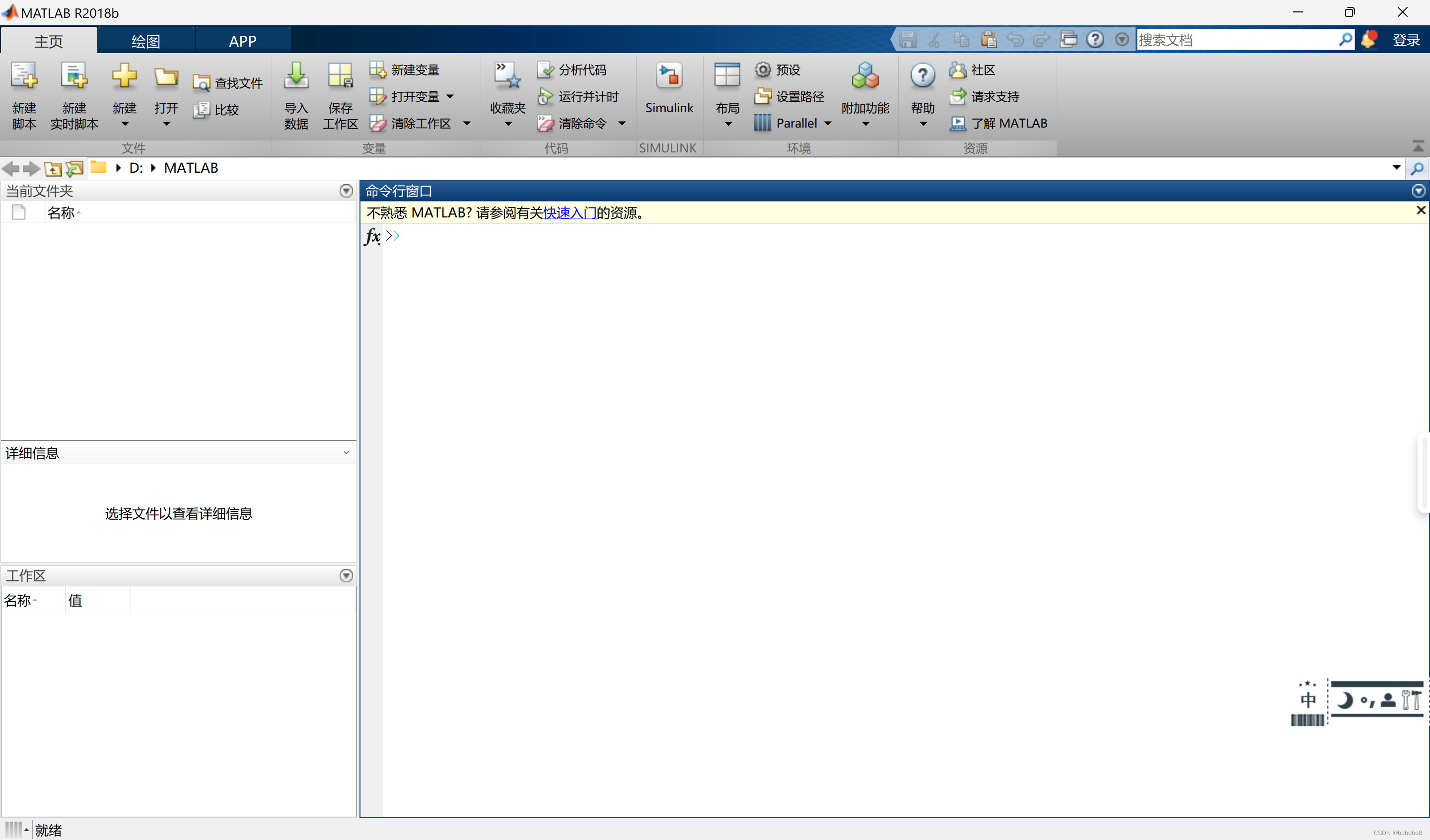
(MATLAB)安装指南
参考链接:MATLAB2019a安装教程(避坑版)...
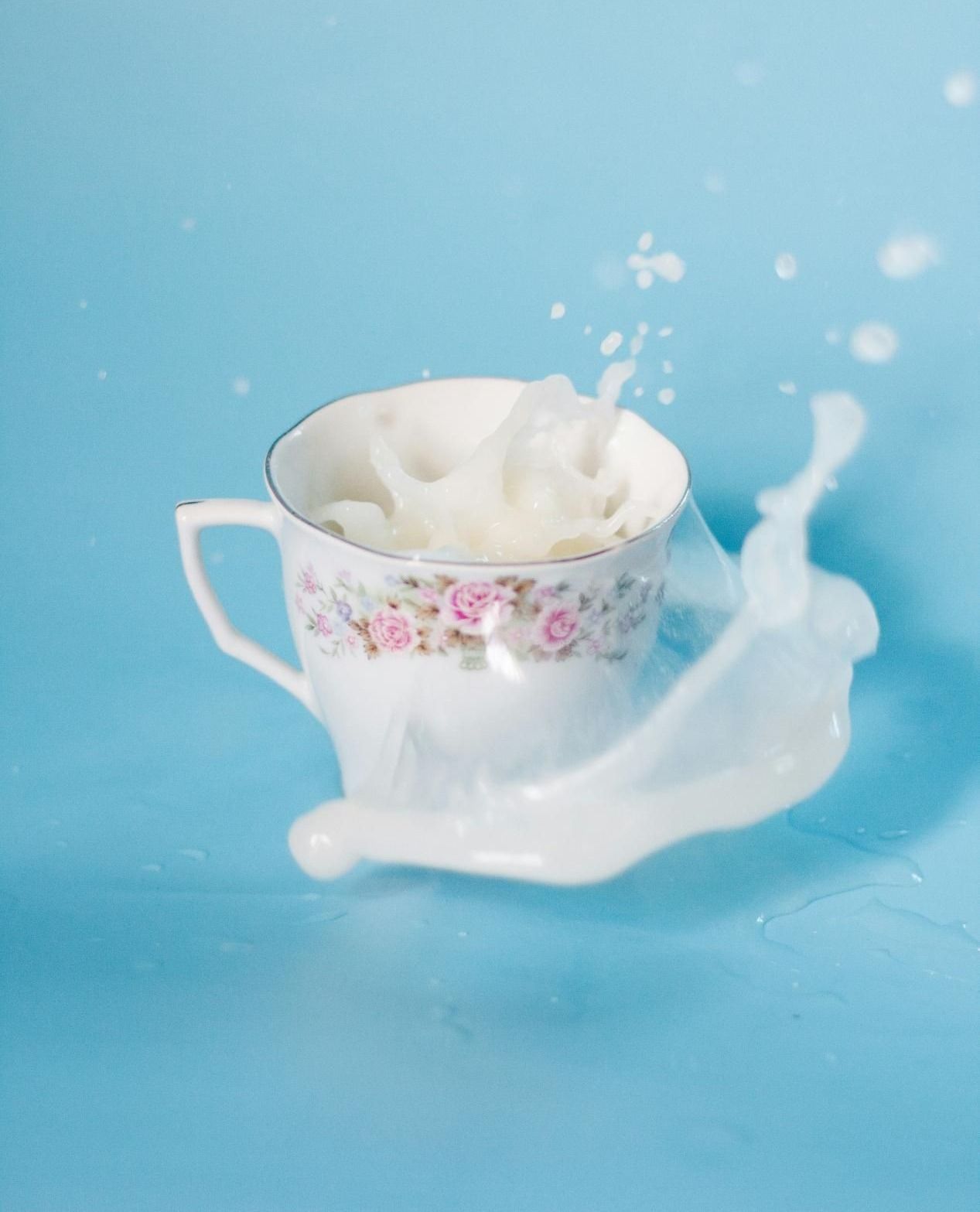
社区智能奶柜:创业新机遇
社区智能奶柜:创业新机遇 在追求高质量生活的今天,健康食品成为大众焦点。社区智能奶柜适时登台,革新了居民获取新鲜牛奶的传统模式,为创业者开辟了一片蓝海市场。 一、新兴创业蓝海:牛奶随享站 日常膳食中…...
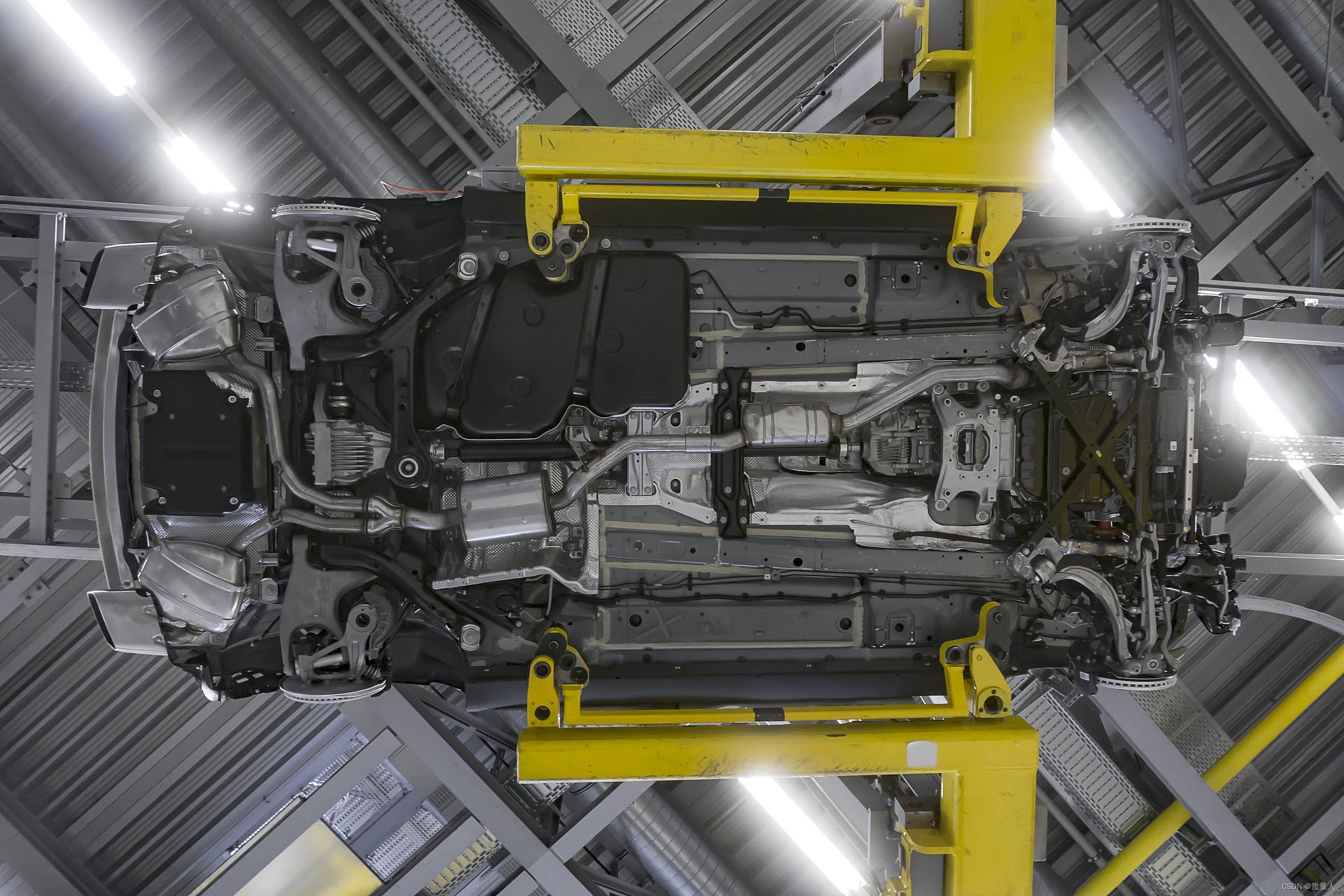
地盘紧固的关键技术——SunTorque智能扭矩系统
底盘紧固件是汽车底盘系统中不可或缺的一部分,它们负责连接和固定各个部件,确保车辆行驶的安全和稳定。底盘紧固件的开发涉及到多个环节和关键技术,下面SunTorque智能扭矩系统将详细介绍底盘紧固件开发流程和关键技术。 一、底盘紧固件开发的…...

Mybatis plus update PG json 类型 报错解决
Mybatis plus update PG json 类型 报错解决 1. 定义的PG数据库对象2. 自定义 JSON Handler3. update Wrapper4. update 报错信息4.1 No hstore extension installed.4.2 Error setting non null for parameter #1 with JdbcType null . Try setting a different JdbcType for …...

精通 Docker:简化开发、部署与安全保障
踏上 Docker 之旅,每一条命令都是高效与可靠的新境界。Docker 彻底改变了软件开发,为构建、部署和保障应用程序提供了前所未有的便利。从打造精益敏捷的镜像到编排复杂的微服务架构,Docker 让开发人员和运维人员都倍感轻松。让我们深入探索 D…...

KIMI的API使用:重点是他的API在使用的适合可以实时调用tool(外部联网等)
User: 如何获取kimi 的API Kimi: 要获取Kimi的API,您需要按照以下步骤操作: 注册账号:首先,您需要访问Kimi开放平台(platform.moonshot.cn/console)并注册一个账号。 获取API Key:登录后,在平台的“账户总览”部分查看平台赠送的免费额度。然后,点击“API Key 管理”…...
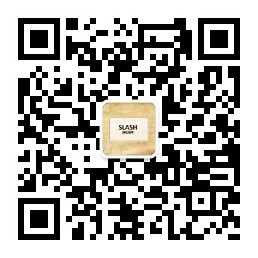
Android内核之Binder读写通信:binder_ioctl_write_read用法实例(七十)
简介: CSDN博客专家,专注Android/Linux系统,分享多mic语音方案、音视频、编解码等技术,与大家一起成长! 优质专栏:Audio工程师进阶系列【原创干货持续更新中……】🚀 优质专栏:多媒…...
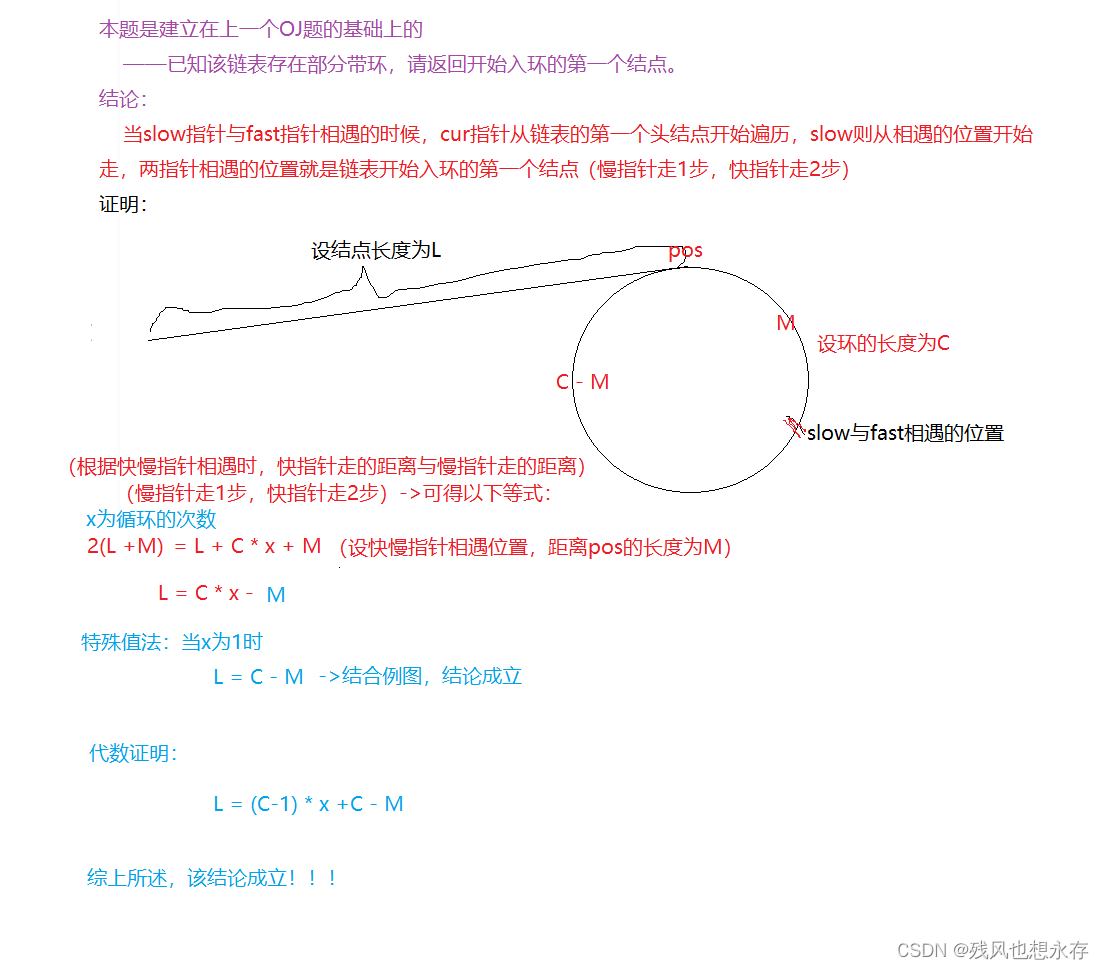
【C语言/数据结构】经典链表OJ习题~第二期——链中寻环
🎈🎈🎈欢迎采访小残风的博客主页:残风也想永存-CSDN博客🎈🎈🎈 🎈🎈🎈本人码云 链接:残风也想永存 (FSRMWK) - Gitee.com🎈…...
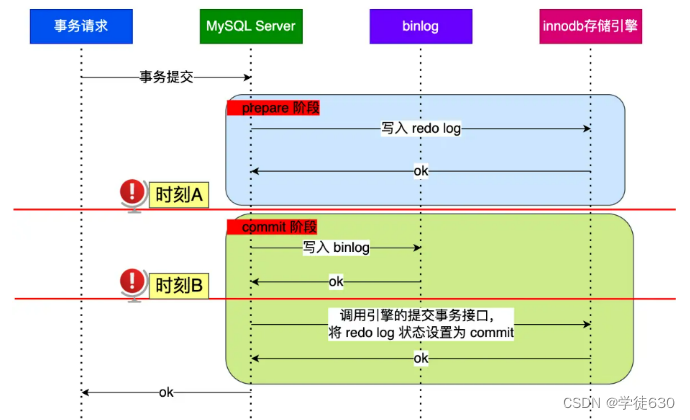
MySQL日志机制【undo log、redo log、binlog 】
前言 SQL执行流程图文分析:从连接到执行的全貌_一条 sql 执行的全流程?-CSDN博客文章浏览阅读1.1k次,点赞20次,收藏12次。本文探讨 MySQL 执行一条 SQL 查询语句的详细流程,从连接器开始,逐步介绍了查询缓存、解析 S…...

SSL通信、证书认证原理和失败原因
目录 SSL通信SSL认证原理SSL证书认证失败的原因分析 SSL通信 SSL通信指的是使用SSL(Secure Sockets Layer)协议进行的加密通讯。SSL是一种标准的安全技术,用于建立一个加密链接,确保从用户的浏览器到服务器之间的数据传输是私密和…...

【MsSQL】数据库基础 库的基本操作
目录 一,数据库基础 1,什么是数据库 2,主流的数据库 3,连接服务器 4,服务器,数据库,表关系 5,使用案例 二,库的操作 1,创建数据库 2,创建…...
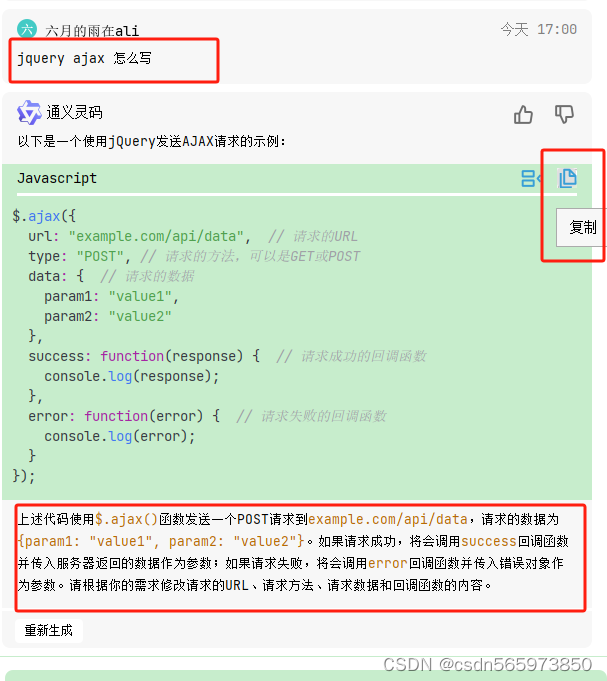
AI编码工具-通义灵码功能实测
AI编码工具-通义灵码功能实测 通义灵码功能介绍行级/函数级实时续写自然语言生成代码单元测试生成异常排错智能排查生成代码注释生成代码解释研发领域自由问答 在上一篇文章中,我介绍了通义灵码的功能以及支持的操作系统,主流IDE等,详细内容可…...

直接显示二进制图片
Option Explicit Private Type GUID Data1 As Long Data2 As Integer Data3 As Integer Data4(7) As Byte End Type Private Declare Function CreateStreamOnHGlobal Lib “ole32.dll” (ByRef hGlobal As Any, ByVal fDeleteOnResume As Long, ByRef ppstr As Any) As Long P…...
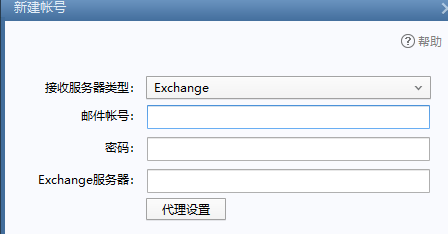
微软exchange邮箱发送
使用java发送exchange类型的邮件,foxmail中配置如下图: 需要的maven依赖如下: <dependency><groupId>com.microsoft.ews-java-api</groupId><artifactId>ews-java-api</artifactId><version>2.0</ve…...
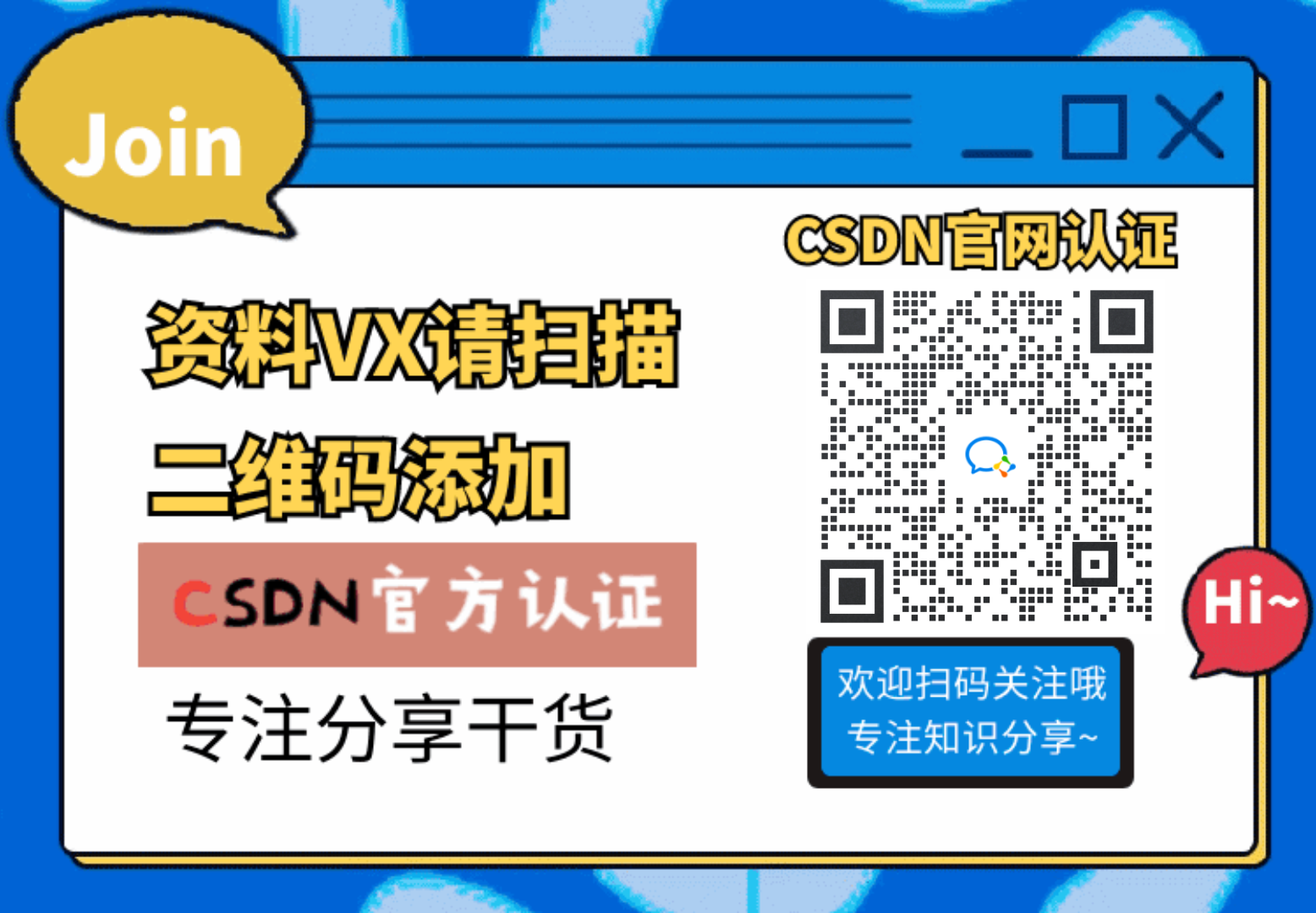
AI绘画Stable Diffusion SDXL 超赞!高质量万能大模型,写实人像、时尚设计、建筑设计、电影制作—筑梦工业XLV4.0
大家好,我是阿威 今天为大家带来了一款多功能大模型——Dream Tech XL | 筑梦工业XL V4.0。该模型是大佬Dr_Dream基于V3.0训练而来的迭代版本,在提升画面质感的同时,对于提示词理解能力有跨越式提升,可以做到100%还原提示词。筑梦…...
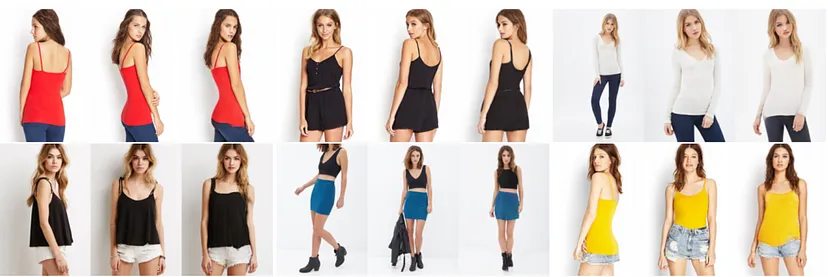
数字人捕捉、建模与合成
在感知系统中,我们与外部合作者一起创建逼真的 3D 人类,其行为可以像虚拟世界中的真实人类一样。这项工作在今天有许多实际应用,并且对于元宇宙的未来至关重要。但是,在感知系统中,我们的目标是科学的——通过重现人类…...

Yarn:下一代JavaScript包管理器的安装与实战指南
当然,让我们深入探讨Yarn——一个高效、可靠的JavaScript包管理器,它为前端开发带来了新的速度和便利。Yarn由Facebook、Google、Exponent和Tilde公司共同推出,旨在解决npm(Node.js包管理器)存在的问题,如依…...

JVM进程缓存 Caffeine
JVM进程缓存 Caffeine 初识Caffeine Caffeine是一个基于Java8开发的,提供了近乎最佳命中率的高性能的本地缓存库。 ben-manes/caffeine: A high performance caching library for Java (github.com) 实例代码 Test void testBasicOps() {// 创建缓存对象Cache&…...
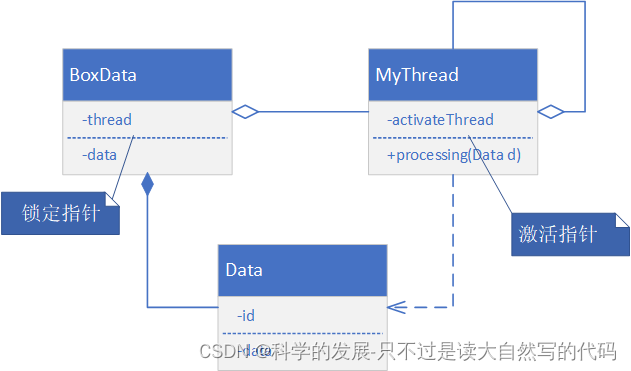
c++ 线程交叉场景试验
1.需求 处理一个列表的数据,要求按照列表的数据处理10个数据可以使用多线程处理,但是针对每个线程,1~10的处理顺序不能变。每个数据的处理必须原子,即只有一个线程可以针对某个数据进行处理,但是10个数据是可以由10个…...

Cell:如何升华你的单细胞数据——PCF空间单细胞蛋白组联合scRNA-seq解析骨髓微环境
骨髓微环境非常复杂,含有不同的细胞类型,包括了造血、间充质、内皮、血管平滑肌和神经谱系细胞等。非造血细胞对于骨髓造血非常关键。然而,这些细胞在人骨髓中的异质性和空间定位在很大程度上仍未被表征。来自佩雷尔曼医学院的研究者使用scRN…...

vue强制刷新组件
在Vue中强制刷新一个组件,通常不是一个推荐的做法,因为Vue的响应式系统设计就是为了自动处理依赖的更新。要强制重新渲染组件,以下是几种方法: 使用key属性: 最常见的方法是改变组件的key属性。当key发生变化时&#x…...
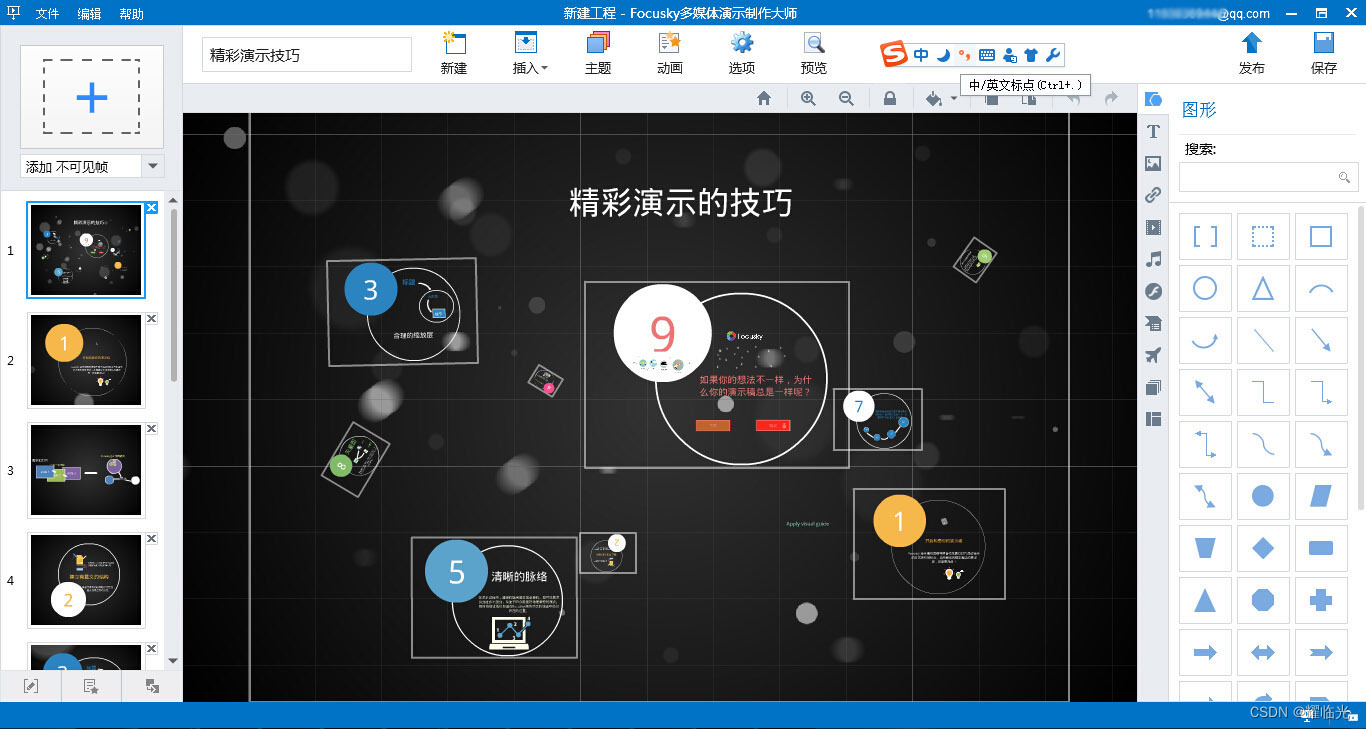
分享5款对工作学习有帮助的效率软件
今天再来推荐5个超级好用的效率软件,无论是对你的学习还是办公都能有所帮助,每个都堪称神器中的神器,用完后觉得不好用你找我。 1.文件复制——ClipClip ClipClip是一款功能强大、操作简便的文件复制与管理软件。它改变了传统的复制粘…...

redis秒杀(PHP版本)
前提提要 今天产品端提了个需求,院校组要求借调我去帮忙,因为我以前做过商城,现在他们需求做一个积分商城,需要做一个秒杀模块,结果毫无意外的我被借调过去了,刚好可以复习一下以前的知识,现在介…...
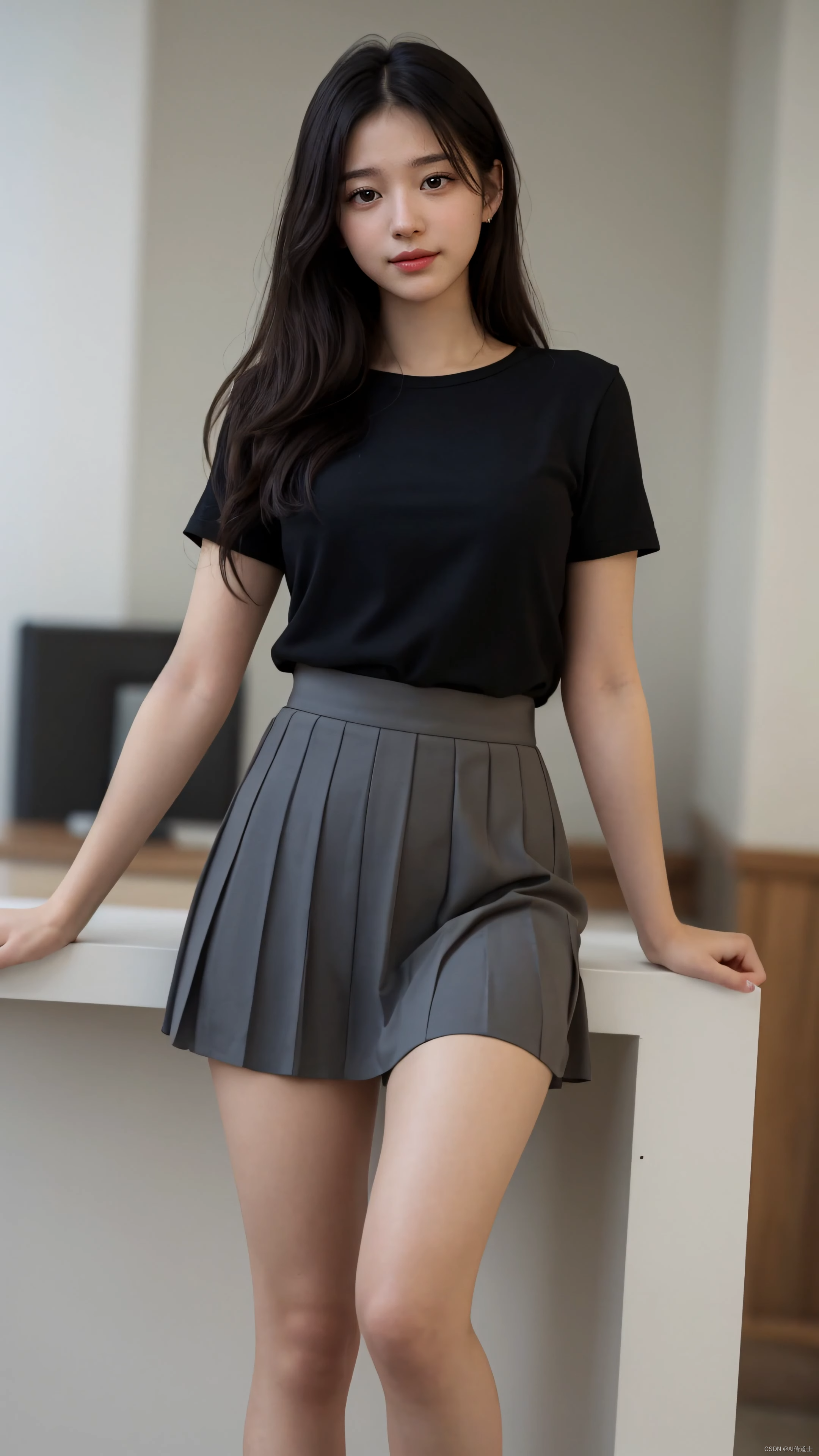
图形用户界面(GUI)在AI去衣技术中的作用与重要性
引言: 随着人工智能技术的不断进步,AI去衣这一概念逐渐进入公众视野。它指的是利用深度学习算法,从图片或视频中自动移除人物的衣物,生成相应的“裸体”图像。尽管这项技术在道德和隐私方面引发了诸多争议,但其背后的技…...
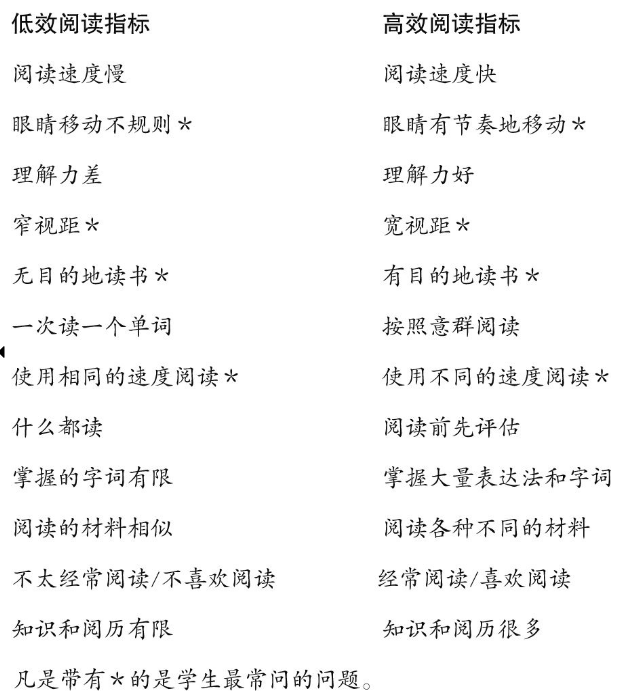
如何阅读:一个已被证实的低投入高回报的学习方法的笔记
系列文章目录 如何有效阅读一本书笔记 如何阅读:一个已被证实的低投入高回报的学习方法 麦肯锡精英高效阅读法笔记 读懂一本书笔记 文章目录 系列文章目录第一章 扫清阅读障碍破解读不快、读不进去的谜题一切为了阅读小学教师让你做,但中学老师阻止你做的…...
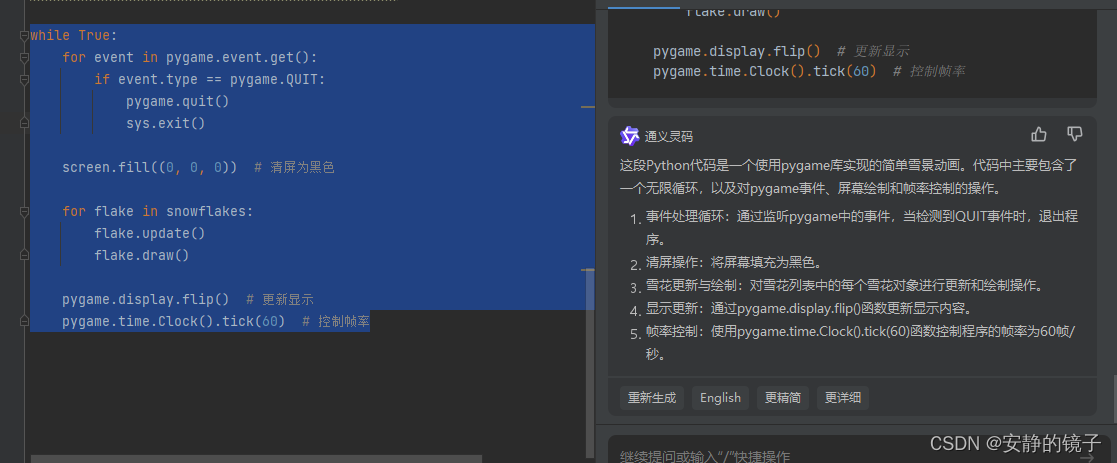
pycharm 安装“通义灵码“并测试
过程:“File>setting>Plugins” 提示: 翻译之后: 点击"接受"之后,提示一下图片,点击ok 安装完成: 安装完"通义灵码"之后,需要登陆,登陆后测试 参考…...

React 之 useMemo Hook (九)
useMemo 是 React 的一个Hook,它允许你“记住”一些计算值,只有在依赖项之一发生变化时才会重新计算这些值。这有助于避免不必要的重新计算和渲染,从而提高应用程序的性能。 代码栗子(计算一个斐波那契数列的值)&#…...
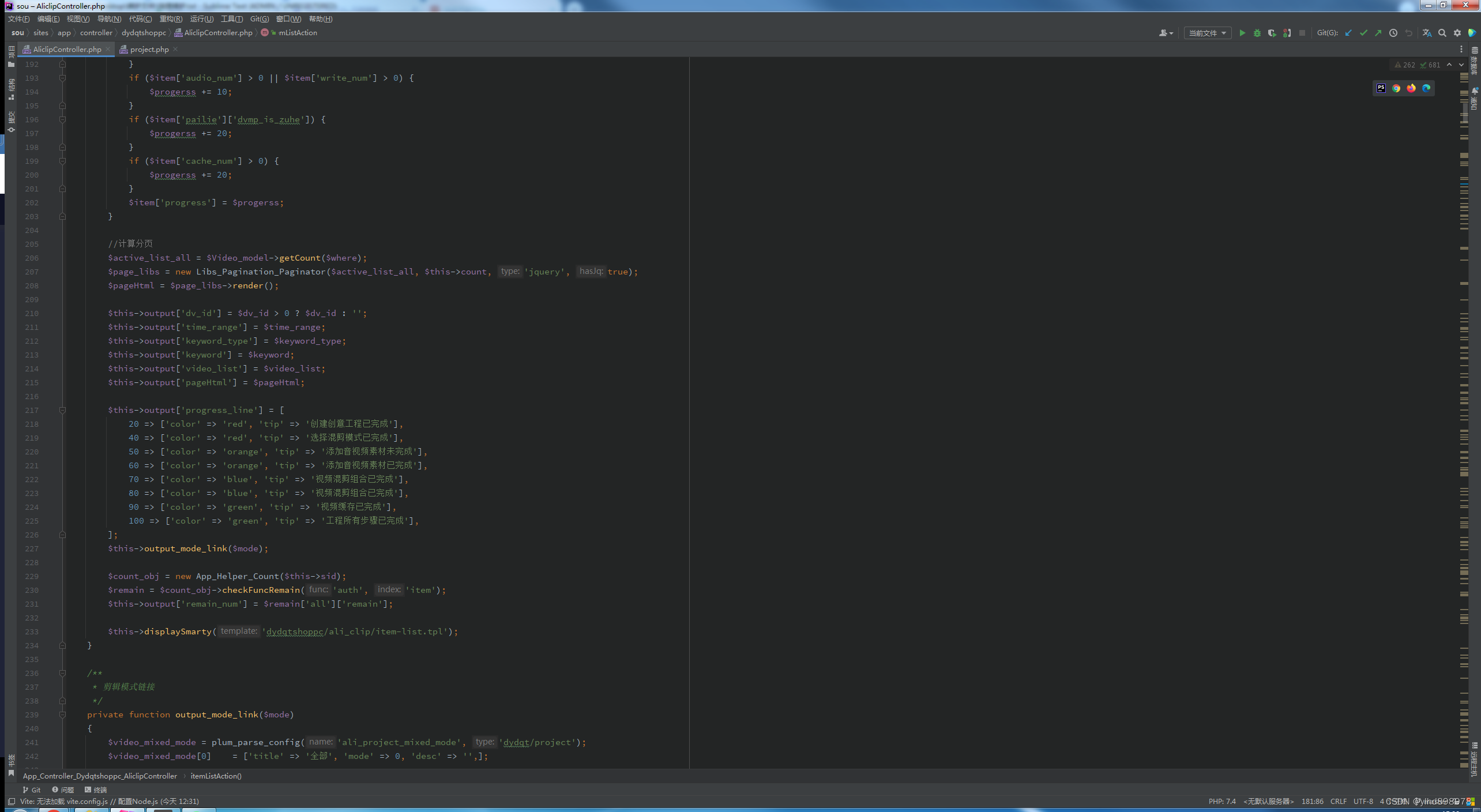
短视频矩阵系统源码saas开发--可视化剪辑、矩阵托管、多功能合一开发
短视频矩阵系统源码saas开发(可视化剪辑、矩阵托管、智能私信聚合、线索转化、数据看板、seo关键词、子账号等多个板块开发) 短视频矩阵系统是一种集成了多种功能的系统,旨在帮助用户在短视频平台上进行高效的内容创作、管理和发布。根据您提…...

百度大模型文心一言api 请求错误码 一览表
错误码说明 千帆大模型平台API包含两类,分别为大模型能力API和大模型平台管控API,具体细分如下: 大模型能力API 对话Chat续写Completions向量Embeddings图像Images 大模型平台管控API 模型管理Prompt工程服务管理模型精调数据管理TPM&RP…...

Unity调用智谱API(简单操作 文本实时翻译)
代码展示: using Newtonsoft.Json; using System; using System.Collections; using System.Collections.Generic; using UnityEngine; using UnityEngine.Networking; using UnityEngine.UI;public class ZhiPuAi : MonoBehaviour {// API的端点URLpublic string…...
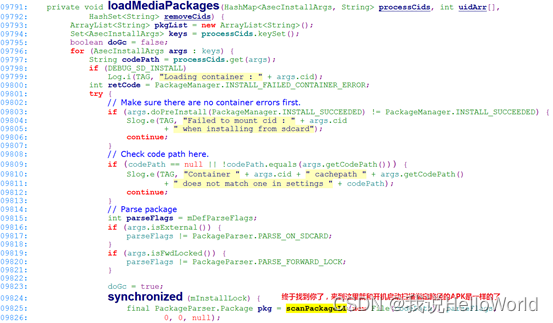
Android 开机启动扫描SD卡apk流程源码分析
在开机的时候,装在SD卡的apk和装在系统盘的apk扫描过程不一样,系统盘apk在系统启动过程中扫描,而SD卡上的就不是,等系统启动好了才挂载、扫描,下面就说下SD扫描的流程: 在SystemServer启动MountService&am…...

如何恢复回收站中被删除的文件?3个恢复策略,实测有用!
“刚刚一不小心把回收站清空了,大家有什么好用的方法可以帮我恢复回收站中删除的文件吗?快帮帮我吧!” 在使用电脑的过程中,我们有时可能会不小心将重要的文件或文件夹删除到回收站,并且随后可能进一步从回收站中彻底删…...
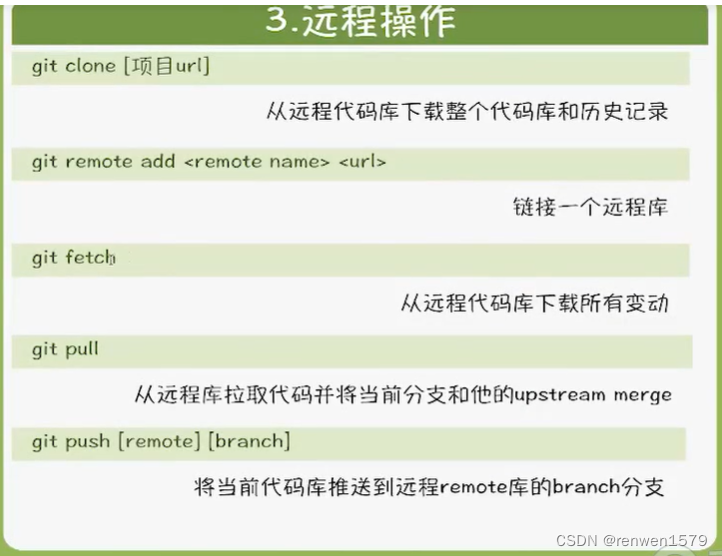
Unity---版本控制软件
13.3 版本控制——Git-1_哔哩哔哩_bilibili Git用的比较多 Git 常用Linux命令 pwd:显示当前所在路径 ls:显示当前路径下的所有文件 tab键自动补全 cd:切换路径 mkdir:在当前路径下创建一个文件夹 clear:清屏 vim…...
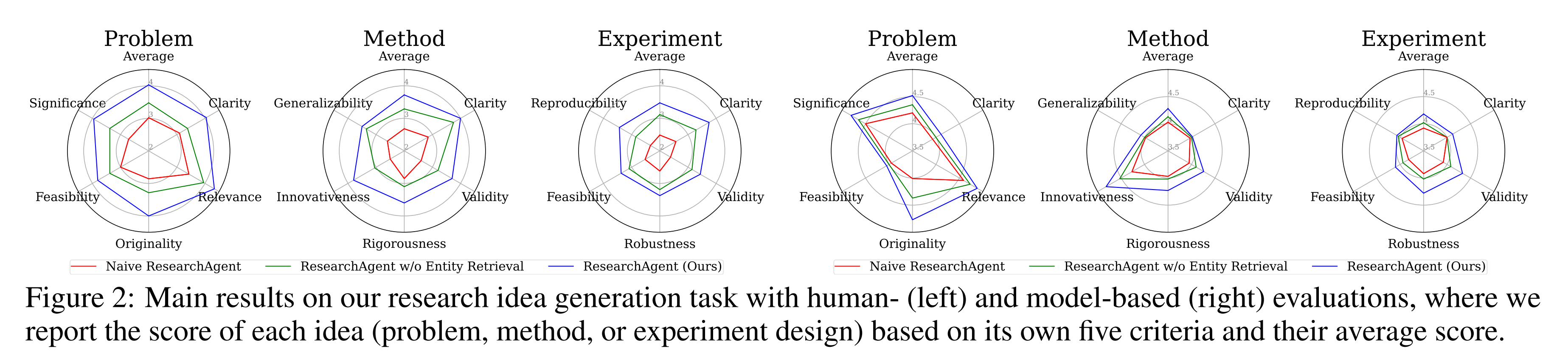
基于大模型的idea提炼:围绕论文和引用提炼idea之ResearchAgent
前言 对本博客比较熟悉的朋友知道,我司论文项目组正在基于大模型做论文的审稿(含CS英文论文审稿、和金融中文论文审稿)、翻译,且除了审稿翻译之外,我们还将继续做润色/修订、idea提炼(包含论文检索),是一个大的系统,包…...

前端深度扩展
1 为什么要有webpack 模块化管理:构建工具支持Common JS、ES6模块等规范;依赖管理:在大型项目中,手动管理文件依赖关系。webpack可以自动分析项目中的依赖关系,将其打包成1个或多个优化过的文件,减少页面加…...
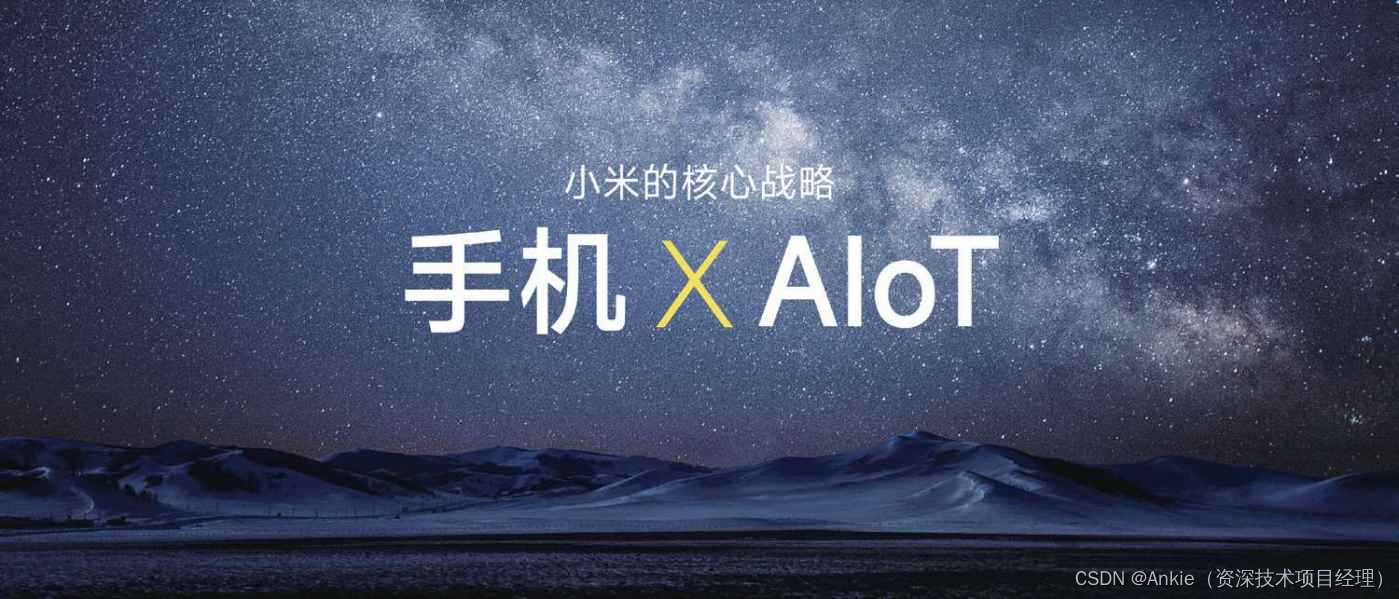
雷军-2022.8小米创业思考-6-互联网七字诀之专注:有所为,有所不为;克制贪婪,少就是多;一次解决一个最迫切的需求
第六章 互联网七字诀 专注、极致、口碑、快,这就是我总结的互联网七字诀,也是我对互联网思维的高度概括。 专注 从商业角度看,专注就是要“把鸡蛋尽量放在一个篮子里”。这听起来似乎有些不合理,大家的第一反应可能是“风险会不会…...
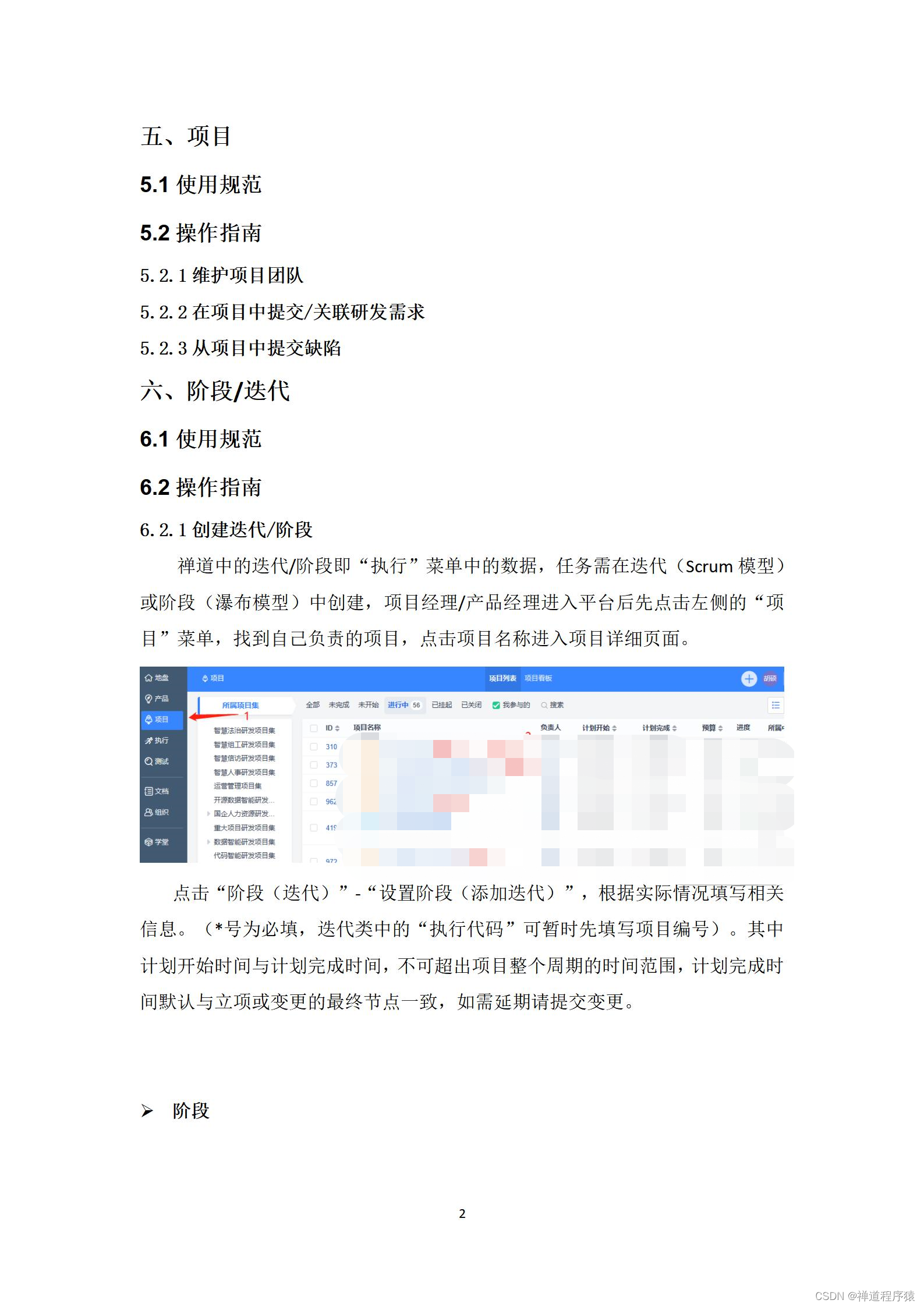
【禅道客户案例】北大软件携手禅道,开启产品化之路新征程
在项目制项目模式下,软件公司根据客户的需求进行短期项目开发,具有灵活、高效、受众面广的优点,在业界得到了广泛的应用。但这种模式也面临诸多挑战,软件公司需要不断地开发新项目来维持业务增长,由于没有自己的产品也…...

解释泛型(Generics)在Java中的用途
在Java中,泛型(Generics)是一种在编译时期提供类型检查和约束的机制,它使得类和接口可以被参数化,即你可以定义一个类或接口,并通过参数传入具体的类型。泛型增加了代码的复用性和类型安全性,同…...
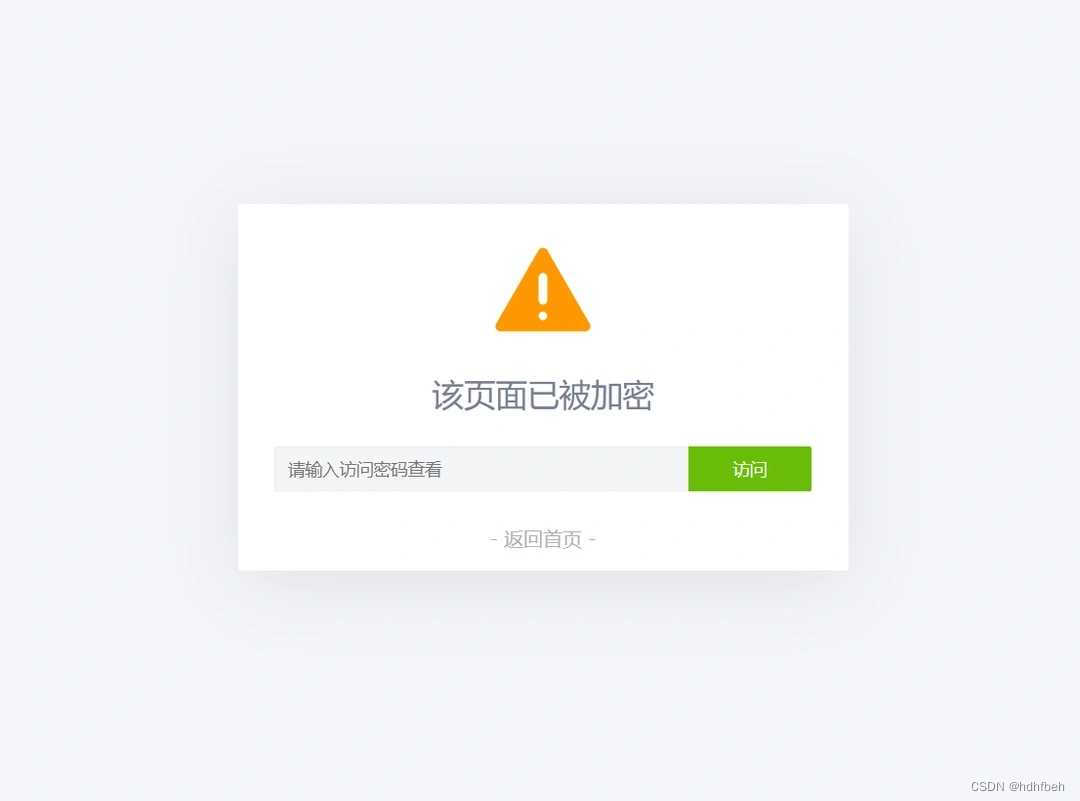
给网站网页PHP页面设置密码访问代码
将MkEncrypt.php文件上传至你网站根目录下或者同级目录下。 MkEncrypt.php里面添加代码,再将调用代码添加到你需要加密的页进行调用 MkEncrypt(‘123456’);括号里面123456修改成你需要设置的密码。 密码正确才能进去页面,进入后会存下cookies值&…...
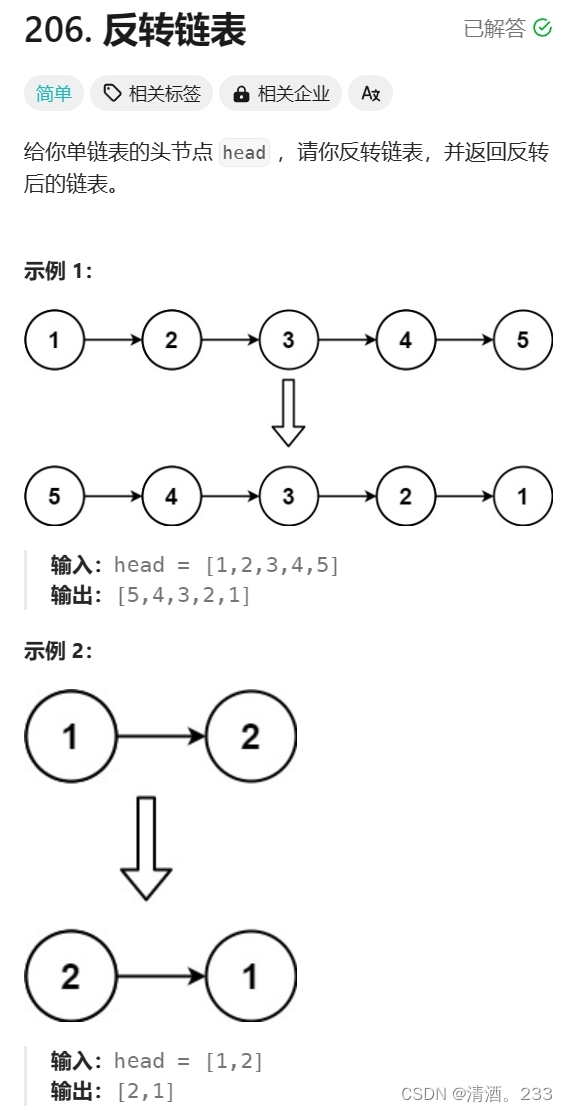
124.反转链表(力扣)
题目描述 代码解决(思路1:双指针) class Solution { public:ListNode* reverseList(ListNode* head) {ListNode*temp;//保存cur下一个节点ListNode*curhead;ListNode*preNULL;while(cur){tempcur->next;// 保存一下 cur的下一个节点&#…...
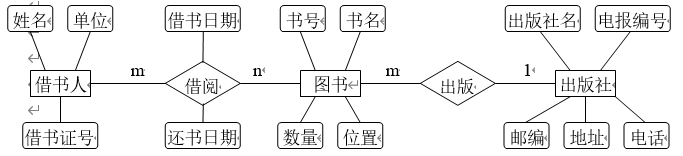
【数据库原理及应用】期末复习汇总高校期末真题试卷06
试卷 一、选择题 1. ________是长期存储在计算机内的有组织,可共享的数据集合. A.数据库管理系统 B.数据库系统 C.数据库 D.文件组织 1. 有12个实体类型,并且它们之间存在15个不同的二元联系,其中4个是1:1联系类型,5…...
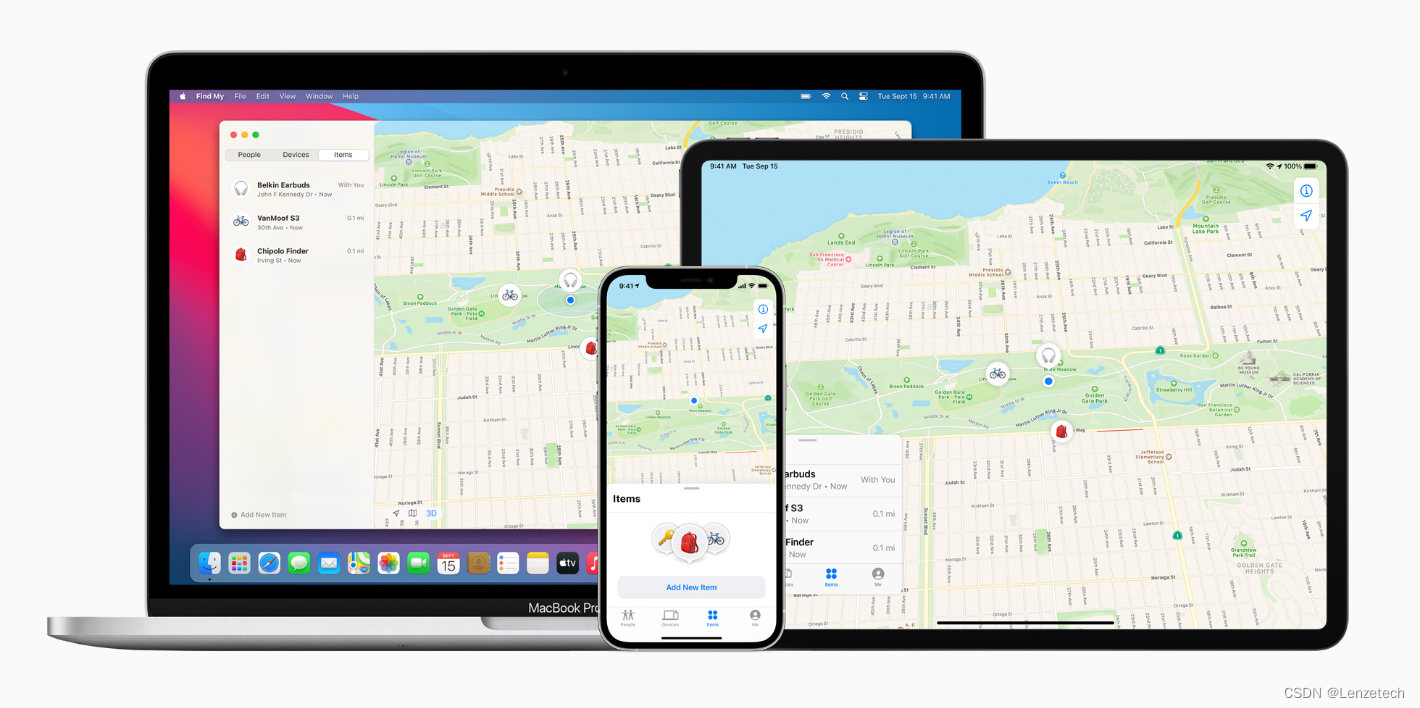
Find My资讯|苹果 iOS 17.5 率先执行跨平台反跟踪器标准
苹果和谷歌公司于 2023 年 5 月宣布推出“检测预期外位置追踪器”(Detecting Unwanted Location Trackers)行业标准,经过 1 年多的打磨之后,该标准目前已通过 iOS 17.5 部署到 iPhone 上。谷歌也将为运行 Android 6.0 或更高版本的…...
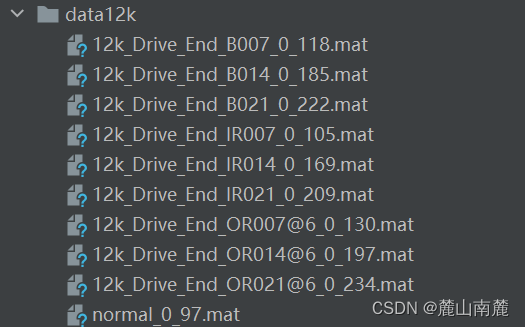
CRWU凯斯西储大学轴承数据,12k频率,十分类
CRWU凯斯西储大学轴承数据,12k频率,十分类。 from torch.utils.data import Dataset, DataLoader from scipy.io import loadmat import numpy as np import os from sklearn import preprocessing # 0-1编码 from sklearn.model_selection import Str…...

Golang面向对象编程
在Go语言中,并没有像传统面向对象编程语言(如Java或C)那样直接支持类和继承的概念。但是,Go通过结构体(struct)和方法(method)来模拟面向对象编程中的对象和行为。 下面是一个简单的…...

Spring的IOC(Inversion of Control)设计模式
Spring的IOC(Inversion of Control)是一种设计模式,它通过控制反转的思想来降低组件之间的耦合度。在Spring框架中,IOC容器负责管理应用程序中的对象,使得对象之间的依赖关系由容器来维护和注入。 以下是Spring IOC的…...
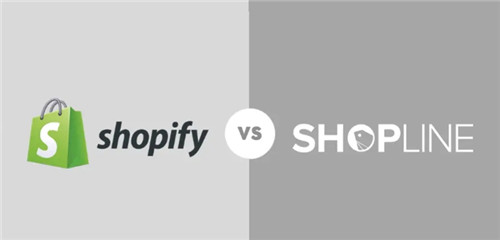
Shopline和Shopify哪个更好?Shopline和Shopify的区别
Shopline和Shopify哪个更好取决于用户面向的市场,面向亚洲市场就更适合有本地化支持的Shopline,而如果希望拓展全球业务,Shopify可能更好。 Shopline和Shopify都是知名的电子商务平台,可以很好的帮助商家搭建和管理在线商店&…...

初步揭开缓存神秘面纱之双map实现缓存管理的类
在应用程序中,缓存是一种常见的优化手段,可以提高数据的访问速度。针对缓存管理,我们通常会实现一些类来方便地管理缓存数据。缓存具体是如何实现的,这里我们利用双map做一个缓存的基本实现。 1.考虑缓存有哪些属性 1.是否是永久…...